Initiation Report: Anyscale - Pioneering Scalable Deployment in Generative AI
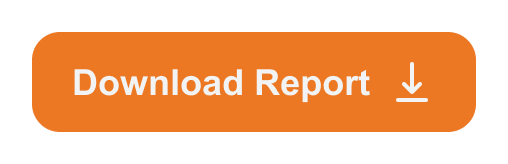
Core Thesis
Generative AI promises transformative impacts across all sectors, yet its full potential is curtailed by critical challenges in scalability, deployment, and expertise. These barriers prevent many organizations from realizing the full value of AI across key applications such as chatbots, virtual assistants, content creation, and language translation. Anyscale addresses these fundamental obstacles, enabling the widespread adoption of generative AI by simplifying its deployment and maximizing its utility.
Methodology
Our views on Anyscale are derived from our rigorous research process, involving proprietary channel checks with users, competitors, and industry experts, and synthesizing publicly available information from the company and other reliable sources.
Key Points
- Technological Leadership with Ray and Anyscale Platform: Anyscale establishes its technological leadership by delivering a remarkable 23x higher throughput and a 75% reduction in costs compared to conventional solutions. Its capacity to scale to 1000 nodes in a mere 60 seconds, alongside setting a world record by shuffling 100TB of data at the cost of just $1/TB, exemplifies its superior performance and efficiency. These capabilities underscore Anyscale’s underlying investment attractiveness in a market that highly values innovation and operational efficiency.
- Cost-Effectiveness Vis-à-vis Proprietary AI Systems: Anyscale offers compelling cost advantages over proprietary AI systems, costing less than half for general workloads and delivering up to 10x savings for specialized tasks. This cost-effectiveness not only makes Anyscale an attractive option for a wide range of applications but could also drive widespread adoption and market share expansion for the company.
- Growing Open-Source Model Adoption to Support Growth: As the LLM market becomes increasingly commoditized, underscored by the proliferation of open-source models, the emphasis shifts from the models themselves to the adaptability of the platforms they run on. Anyscale is poised to harness this shift with its model-agnostic infrastructure that resonates with developers’ growing need for tools that seamlessly integrate diverse models with unique data sources and application logic. This strategic alignment with market trends enhances Anyscale’s investment appeal in a landscape valuing flexibility and open- source innovation.
- High Scalability Across Industries Will be Growth Multiplier: Anyscale’s scalability is a critical driver for its growth, enabling the platform to handle increasing demands seamlessly across various industries and applications. This flexibility attracts a diverse customer base, from startups to large enterprises, across various sectors. By promoting efficient resource utilization, essential for data-heavy industries, its architecture reduces costs and increases operational efficiency.
- Anyscale’s Open-Source Focus – a Double Edge Sword: Anyscale’s growth strategy is closely tied to the burgeoning adoption of open- source models. While this reliance positions the company at the forefront of industry innovation, it also exposes it to the volatility of market preferences and technological trends.
- Dedicated AI Inference Chips to Intensify Competition: The emergence of companies specializing in dedicated AI inference chips, exemplified by Groq’s significant achievements, represents a growing investment concern for Anyscale. These firms are rapidly advancing in delivering high-throughput, cost-effective solutions, challenging Anyscale’s competitive edge.
- Poised for Upward Valuation Revision: Anyscale’s last post-money valuation stood at $1 billion as of December 2021 with an impressive 447% valuation jump from Series B to C, highlighting strong investor confidence and attesting to its burgeoning potential. Furthermore, Anyscale’s impressive roster of key clients—including industry juggernauts like Uber, OpenAI, AWS, Cohere, Ant Group, Instacart, and Spotify—serves as a robust testament to its value proposition and market acceptance. Considering the rapid growth tive AI adoption and the pivotal role that Anyscale is anticipated to play in this expanding market, its value proposition is poised to soar.
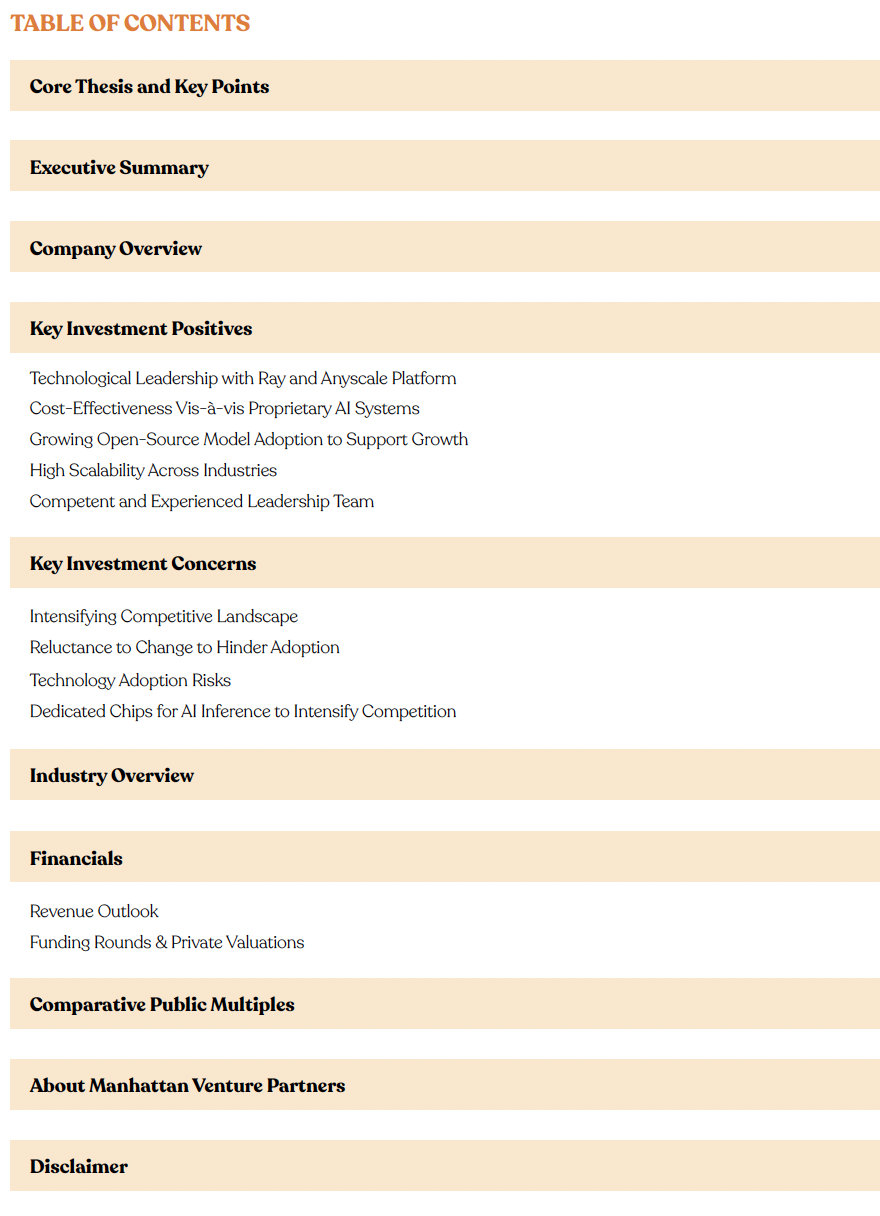
Executive Summary
Since 2012, the computational demands of AI training have increased exponentially with a 3.4-month doubling time, outpacing Moore’s Law (by comparison, Moore’s Law had a 2-year doubling period). This remarkable expansion underscores a seismic shift in the computa- tional landscape and necessitates a platform capable of not only keeping pace but also simplifying the complexities of scaling AI/ML and Python applications for production deployment. Enter Anyscale.
Anyscale has emerged as the go-to solution to the most fundamental challenge confronting AI development teams today: scaling AI/ML and Python applications and deploying them in production. The Anyscale platform, underpinned by Ray open-source framework, offers a seamless solution for the scalable deployment of AI applications. Ray’s ease of integration transforms the development landscape, en- abling developers to effortlessly scale applications from prototype to production.
Anyscale’s cost-saving potential is a significant draw for investors and clients alike, particularly when compared to proprietary AI systems. The growing adoption of open-source models and Anyscale’s ability to provide high scalability across various industries bolster the com- pany’s market position. Anyscale Services enhances this value proposition by reducing operational costs and ensuring reliable, efficient resource utilization in production.
Evidenced by its adoption by industry leaders such as OpenAI, Instacart, and Uber, and supported through partnerships with tech giants like Nvidia, Anyscale’s impact is significant and far-reaching. From ChatGPT to Spotify recommendations to Uber ETA predictions, top companies use Anyscale and Ray to power their solutions. The substantial 447% surge in Anyscale’s valuation between Series B and C, bolstered by investment from industry heavyweights such as Intel Capital and Andreessen Horowitz, reflects a resounding endorsement of its strategic position within the AI development landscape.
Company Overview
Established in 2019, Anyscale provides a platform for scaling compute-intensive machine-learning workloads. Boasting a prestigious user base including LinkedIn, Amazon, Uber, OpenAI, and Visa, the platform’s credibility is indisputable. Anyscale’s Ray stands out as the fastest-growing open-source project in distributed AI, amassing an impressive 18,000 GitHub stars. The startup has garnered support from top-tier investors such as Addition, Andreessen Horowitz, and Intel Capital, solidifying its credibility. With a remarkable 447% valu- ation increase from Series B to C, investor confidence underscores the company’s promising potential. Noteworthy collaborations with industry giants NVIDIA and Meta further enhance Anyscale’s technological prowess. Positioned strategically to capitalize on the surging compute demands in the generative AI revolution, the platform’s user-friendliness serves as a key competitive advantage.

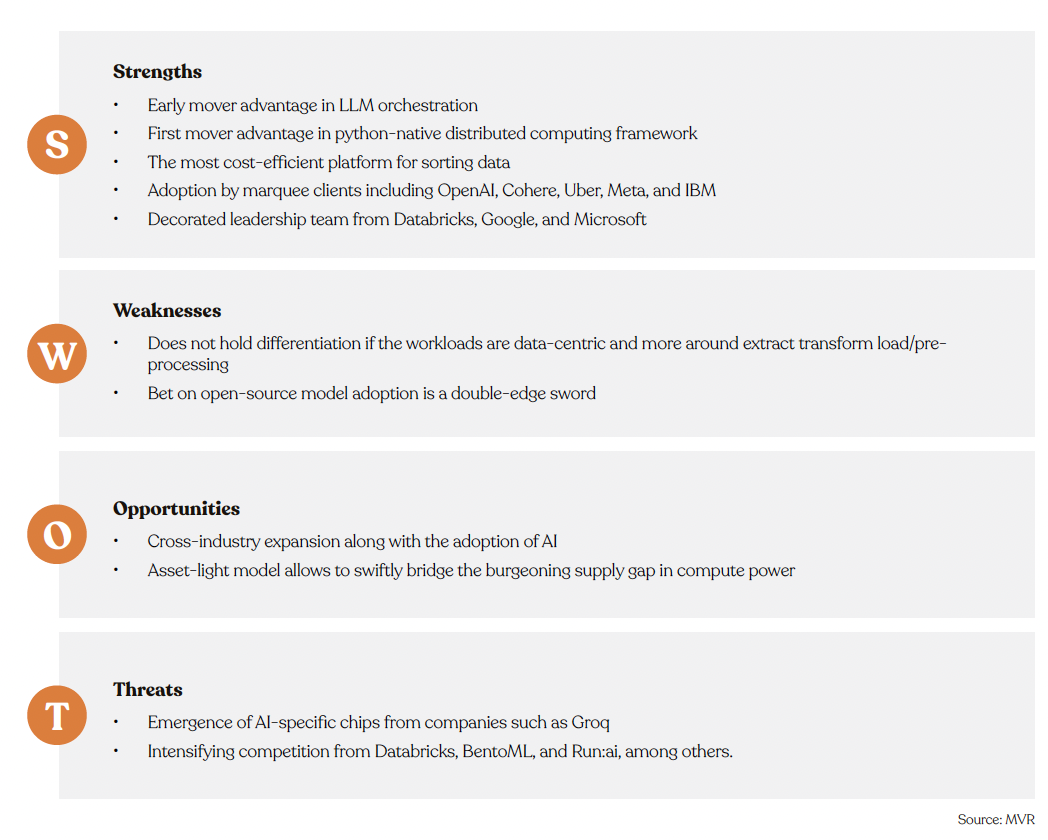
Anyscale: Key Products
Anyscale’s portfolio includes 4 offerings – Anyscale Endpoints, Anyscale Private Endpoints, Anyscale Platform, and Ray Open Source.
Anyscale Endpoints
Anyscale Endpoints is at the forefront of simplifying the deployment, fine-tuning, and scaling of LLMs through production-ready APIs. Designed for developers and businesses seeking to leverage the power of LLMs without the complexities of infrastructure management, Anyscale Endpoints offers a fast, cost-efficient, and serverless solution to bring cutting-edge AI capabilities into applications.
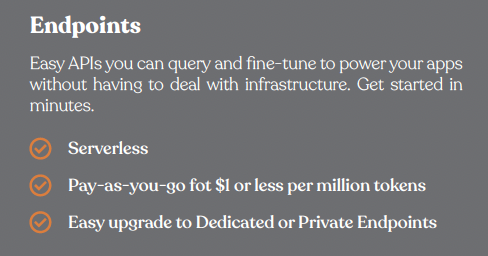
The imminent evolution of this product, termed Dedicated End- points, represents further advancement. This extension will provide dedicated GPU resources, enabling clients to seamlessly deploy custom models and efficiently scale their applications.
Pricing at a competitive rate of $1 or less per million tokens, clients gain access to Anyscale’s expanding roster of high-performance models or the option to deploy their proprietary models. This cost-effective solution underscores Anyscale’s commitment to providing unparalleled value while facilitating optimal performance and scalability for clients.
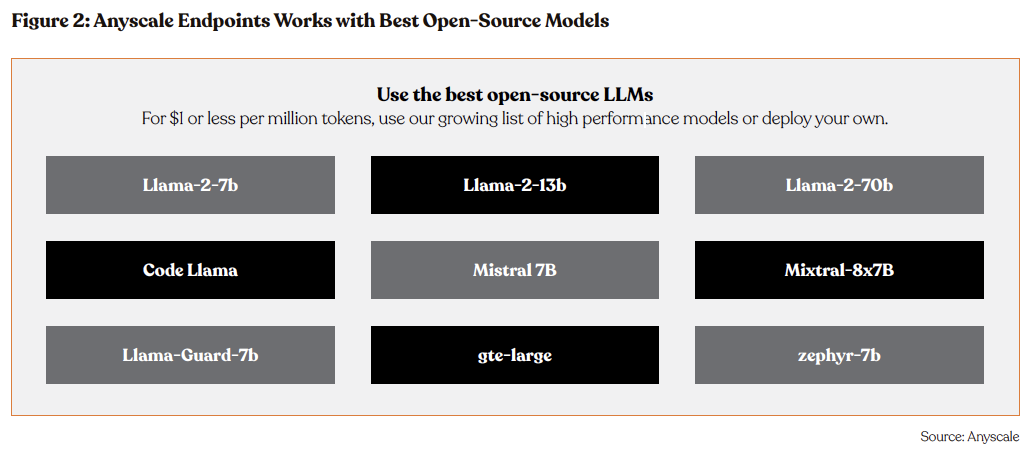
Anyscale Private Endpoints (Coming Soon)
Anyscale Private Endpoints redefine the integration of LLMs with enterprise-grade features, offering a full-stack LLM API solution that operates within the client’s cloud environment. The platform provides enterprise-level security, supplemented by dedicated technical support, Model Refinement/Optimization teams, and customer success services, all facilitated by th d creators of Ray. This holistic approach ensures each client maximizes the value of LLMs tailored to their specific use cases.
Key Product Features
- Full-Stack API Solution in Cloud
- Built for Enterprise
- Optimized Performance
- Ease of Deployment and Operations
- Complete Control
- Seamless Integrations and Simple APIs
- Advanced Security Measures
- Dedicated Support and Services
Anyscale Platform
The Anyscale Platform stands out as the premier enterprise-ready, fully managed solution built upon the Ray ecosystem, designed to supercharge the lifecycle of AI/ML and Python workloads from development through deployment. This pioneering platform transcends the capabilities of Ray, offering enhanced features to expedite the development, deployment, and management of Ray applications in a production setting. Organizations leveraging the Anyscale Platform experience accelerated time-to-market and streamlined iterations across the entire AI lifecycle, underscoring its effectiveness in enhancing operational efficiency.
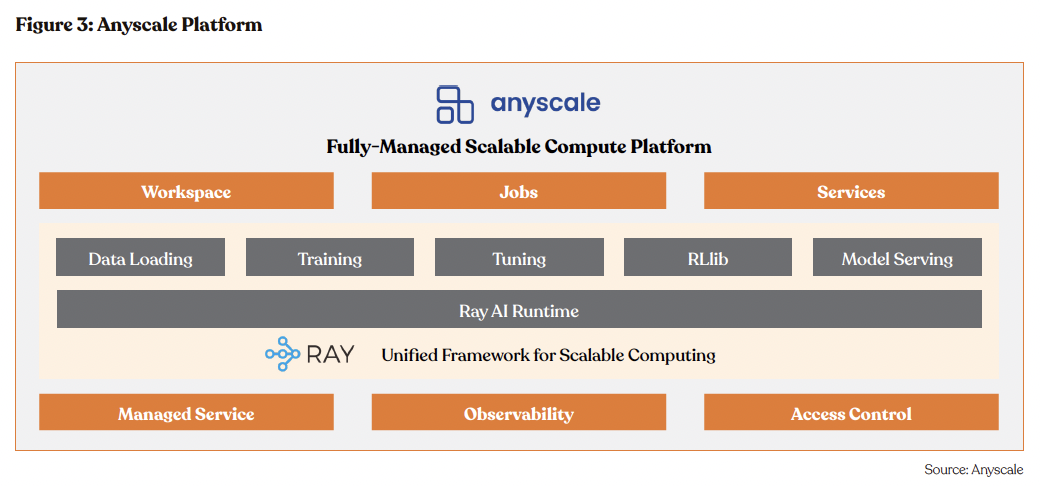
Key Product Features
- Enterprise-Ready: Tailored for businesses, it’s the first of its kind to offer a fully managed Ray environment that’s ready for enterprise deployment.
- Streamlined Development: With Anyscale Workspaces, developers are provided an integrated environment that fosters quick development, collaboration, and effortless model deployment.
- Fully Managed Ray Clusters: Anyscale eliminates the overhead of complex infrastructure management, freeing developers from the need to maintain computing clusters.
- Focus on Innovation: By simplifying the operational side of Ray applications, Anyscale allows developers to dedicate their time to creating impactful, user-centric applications.
- Built-in Monitoring: Integrated observability tools ensure performance is transparent and optimized throughout the application lifecycle.
- Secure and Compliant: The platform includes comprehensive security features, ensuring enterprise-grade protection for your AI workloads.
Ray Open Source
Ray merges the capability to handle highly compute-intensive machine learning tasks with the ease of development akin to working on a laptop. It covers the entire AI lifecycle, from data ingestion and model training to hyperparameter tuning and deployment, all on a single scalable compute platform. This integration empowers developers to design their applications on a laptop and then seamlessly scale up to run on hundreds of machines without any modifications to the code. With its robust ecosystem of distributed machine learning libraries, Ray facilitates scaling across various stages of the development process. It’s the fastest expanding open-source project in distributed AI, boasting 18,000 GitHub stars and adoption by thousands of organizations worldwide, including industry giants like Amazon, Ant Group, LinkedIn, Shopify, Uber, and Visa.
Being open-source and Python-native, Ray offers unparalleled flexibility, allowing easy integration of any ML library or framework into applications. This adaptability is further enhanced by Ray’s compatibility with various hardware accelerators, including GPUs and TPUs, enabling developers to optimize their applications for diverse computing environments. Its credibility is underscored by notable adoptions from industry leader e, solidifying its position as a trusted platform for handling the world’s most demanding AI workloads.
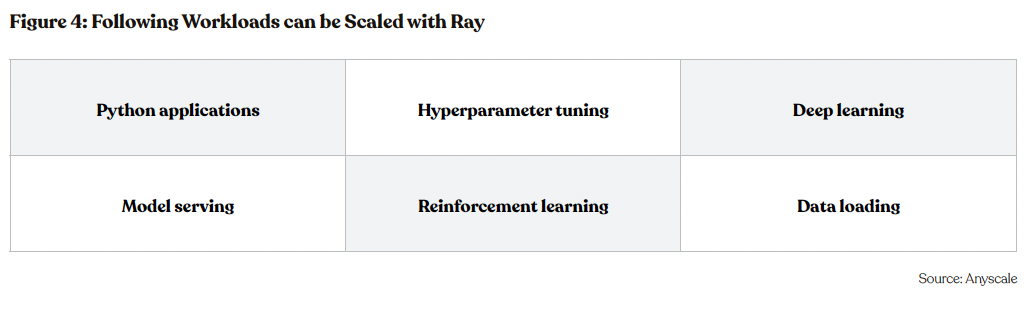
Key Investment Positives
Technological Leadership with Ray and Anyscale Platform: Ray stands out for its technological supremacy, offering a sophisticated computing framework that significantly amplifies the scalability of AI/ML and Python applications. This framework enables developers to effortlessly develop and scale their applications, tackling key obstacles in distributed computing like scalability challenges and user- friendliness. As a pivotal resource for enterprises managing extensive data processing tasks and AI-driven initiatives, Ray underscores the technical excellence and innovation at the heart of Anyscale’s offerings, signaling its capacity to dominate this specialized market segment.
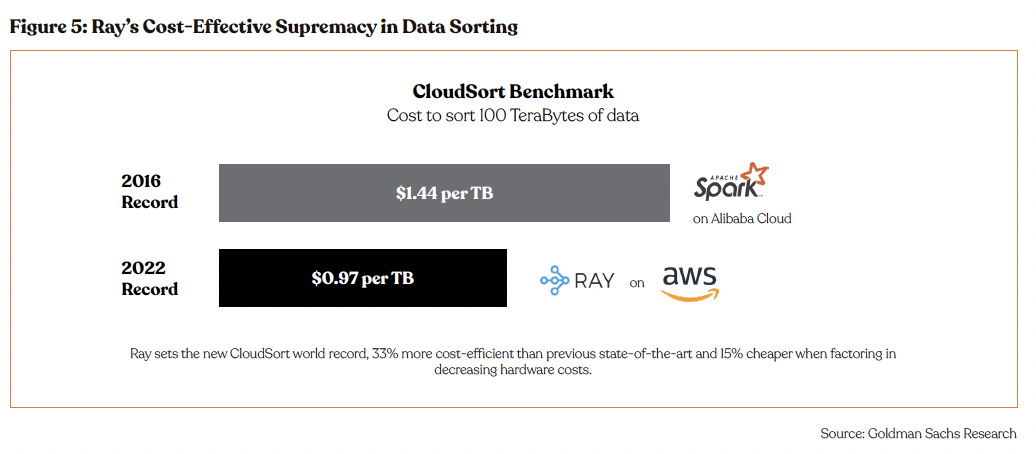
Additionally, Ray’s operational scalability enables its users to start with single-node tasks and seamlessly progress to complex, multi-node operations. On one node, Ray excels in fine-tuning models and executing local predictions, supported by thorough documentation that eases initial use and future expansion. This approach equips developers with a user that’s not only accessible for early-stage development but is also primed for scaling to more demanding workloads.
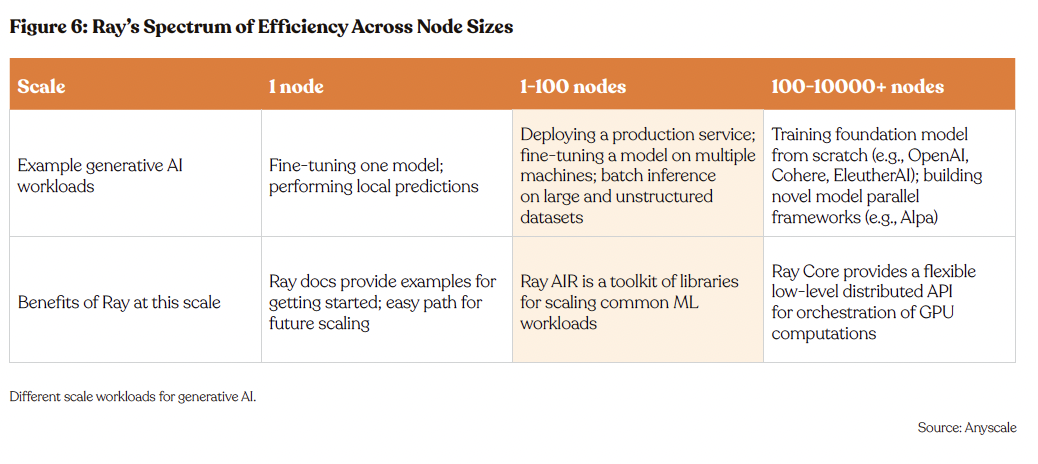
In environments utilizing 1-100 nodes, Ray showcases its AI Runtime (AIR), a comprehensive library suite that simplifies the scaling of machine learning tasks. This functionality is crucial for deploying production services and facilitating batch inf on large, unstructured datasets, making Ray an attractive proposition for businesses seeking to amplify their AI capabilities.
"We looked at a half-dozen distributed computing projects, and Ray was by far the winner. … We are using Ray to train our largest models. It’s been very helpful to us to be able to scale up to unprecedented scale. … Ray owns a whole layer, and not in an opaque way" - Greg Brockman, President, Chairman & Co-Founder, OpenAI
Ray's Seamless Integration Across the ML and Data Ecosystem
Ray’s comprehensive integration features underscore its indispensable value in simplifying and accelerating the deployment of complex ML and data processing tasks. Ray integrates with the entire ML and data ecosystems, including deep learning frameworks (e.g., Tensor- Flow, PyTorch), traditional machine learning libraries (e.g., scikit-learn), workflow orchestration platforms (e.g., Airflow, Perfect), experi- mental tracking (e.g., MLflow, Weights & Biases), features stores (e.g., Databricks, Feast, Hopsworks), and model registries (e.g., Databricks, SageMaker). Ray’s adaptability extends further to accommodate various data formats and systems including Delta Lake and Snowflake, showcasing its extensive reach and adaptability.
At its core, Ray is Python-native, simplifying the scaling process for Python and ML projects to just a handful of code lines or the straight- forward application of Ray AIR libraries. This inherent compatibility allows for the seamless use of favored Python libraries across a wide spectrum of functions from deep learning, statistical analysis, to natural language processing and beyond.
Anyscale enhances Ray’s integration capabilities by streamlining the connection process with widely-used tools, ensuring effortless au- thentication and fostering robust, two-way interactions between Ray and other key platforms. This strategic capability not only under- scores Ray’s role in driving technological innovation bus also solidifies its position as a vital component for enterprises aiming to harness advanced AI and ML technologies efficiently.
Ray's First of its Kind in AI
Against the backdrop of established distributed systems like Apache Spark, Apache Kafka, and Apache Flink, which have been instrumental in scaling data-intensive applications, Ray emerges as a disruptor. These traditional platforms, rooted in Java, primarily focus on optimizing data processing workflows. In stark contrast, Ray introduces itself as a groundbreaking, Python-native distributed computing framework. Its unique selling point lies in its ability to perform with equal efficiency, whether on a solitary local machine or across an expansive network of hundreds of machines.
At the heart of Ray’s cutting-edge strategy is Ray Core, a key element of the Ray ecosystem. Ray Core brings forth straightforward primitives that simplify the task of parallelizing Python applications, enabling developers to scale their projects seamlessly from personal development environments to expansive cloud-based clusters. Further enhancing Ray’s appeal is the Ray AI Runtime (AIR), which pro- vides machine learning-specific support for a range of operations, including hyperparameter tuning, reinforcement learning, and model deployment in production settings. This dedicated focus on machine learning processes highlights Ray’s innovative contributions to the domain, positioning it as an essential resource for AI research and development.
Ray’s dedication to Python and its endeavor to ease the transition from development to deployment in AI initiatives distinctly position it within the distributed computing arena. This strategic orientation ensures that Ray transcends the capabilities of a mere distributed system to become a pioneer in enabling machine learning and AI development at scale. Offering a harmonious mix of simplicity, scalability, and specialization, Ray stands unparalleled by conventional systems that are tailored more towards data processing tasks.
Scalable Model Serving with Ray Serve
Ray Serve stands out as a versatile model serving solution designed for the scalable deployment of online inference APIs. Its frame- work-agnostic nature allows for the seamless serving of a wide array of machine learning models—from deep learning frameworks like PyTorch, TensorFlow, and Keras to Scikit-Learn and custom Python logic. Ray Serve is finely tuned for LLMs, boasting features such as response streaming, dynamic batching, and multi-node/multi-GPU serving capabilities, ensuring high performance and efficiency.
Ray Serve simplifies the development and deployment process, merging simplicity, flexibility, and scalability. This dual approach not only helps reduce operational costs and maximize hardware efficiency but also offers a Python-centric development experience, ideal for both local development and testing. Additionally, Anyscale Services enhances Ray Serve’s capabilities by managing deployment infrastructure and integrations. Heavily production-tested, it guarantees reliability in production, allowing for the creation of innovative AI applications without the burden of infrastructure maintenance. Anyscale Services further optimizes this ecosystem with hardware enhancements to lower costs and ensure greater GPU availability.
Moreover, Ray Serve excels in model composition and multi-model serving, enabling the development of complex inference services that integrate multiple ML models and business logic in Python. This positions it as an optimal choice for crafting sophisticated, AI-powered applications.
Leveraging Ray’s underlying infrastructure, Ray Serve offers unparalleled scalability and efficient resource management, including frac- tional GPU support. This ensures cost-effective scaling of machine learning models, affirming Anyscale’s commitment to providing advanced, accessible computational solutions. Ray Serve not only underscores Anyscale’s technological leadership but also its strategic vision in optimizing resource utilization and reducing operational overhead, effectively addressing the critical challenges in deploying AI technologies.
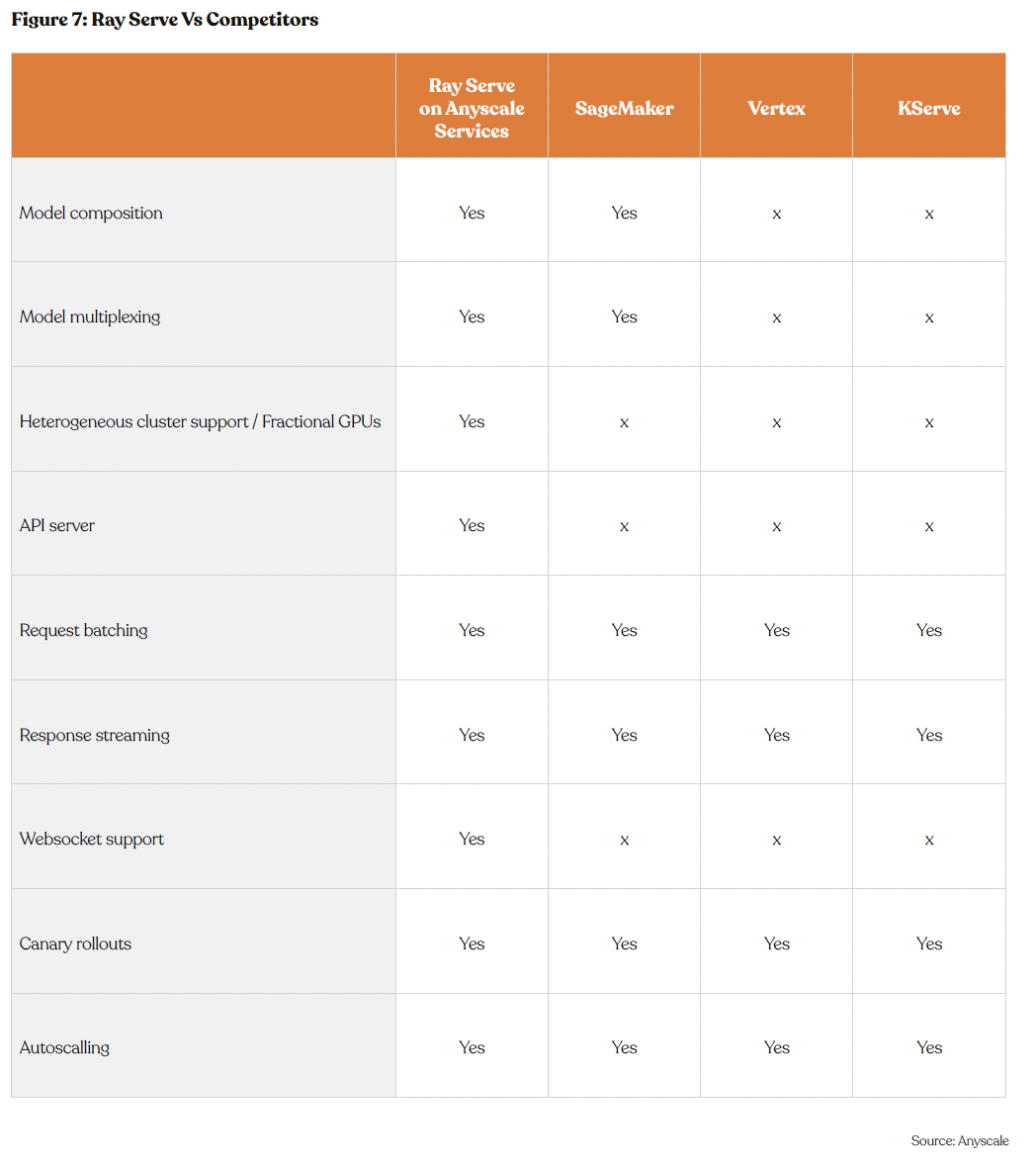
Anyscale - Leading Inference Wars
Anyscale’s holds technological edge in optimizing live-inference workloads for LLMs through advanced continuous batching techniques, outperforming traditional static batching frameworks on key metrics of throughput and latency. Utilizing a single NVIDIA A100 GPU and Meta’s OPT-13B model, a study compares several frameworks, including Hugging Face’s Pipelines and FasterTransformer (static batching) against more advanced continuous batching solutions like Hugging Face’s text-generation-inference, Continuous batching on Ray Serve, and UC Berkeley’s vLLM. The findings highlight continuous batching’s superior ability to handle variable sequence lengths efficiently, doubling performance and significantly reducing latency across all percentiles compared to static approaches.
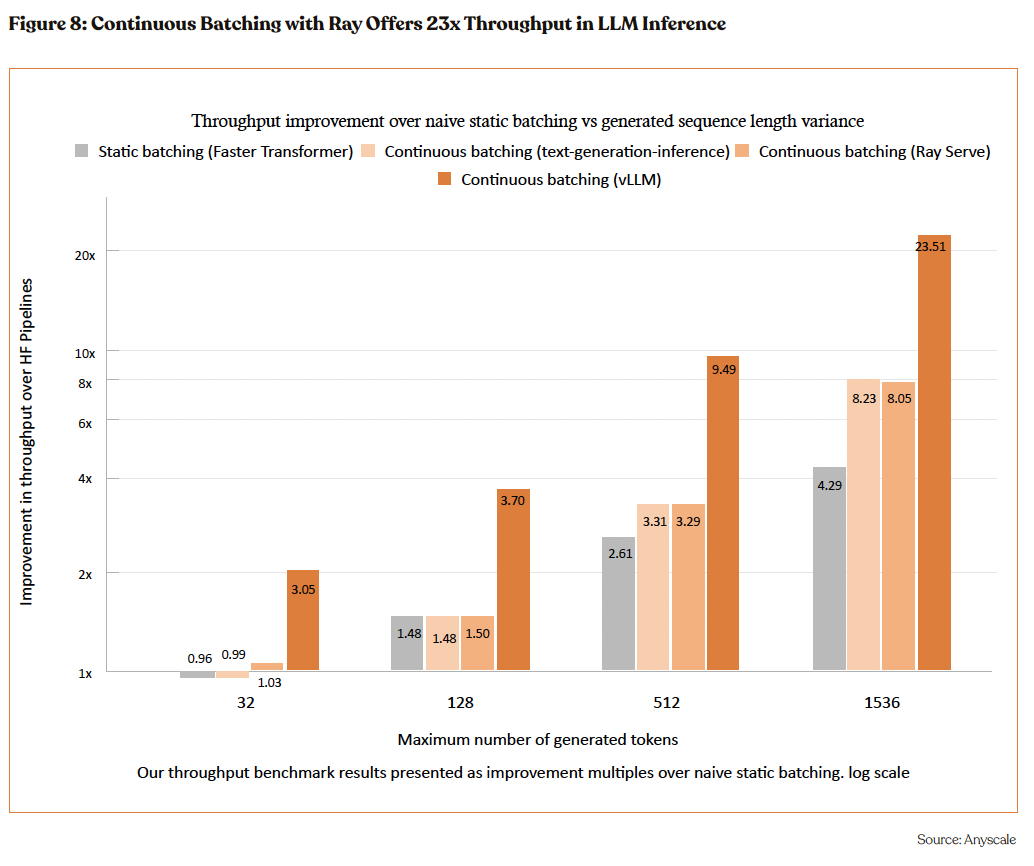
Particularly, vLLM demonstrates remarkable improvements in memory optimization and scheduling, ensuring low latency even under high query rates. This benchmarking underscores Anyscale’s leading position in delivering cost-effective, scalable, and responsive AI services, emphasizing its potential for innovation and market leadership in the AI and machine learning sector, crucial insights for investors evaluating the company’s market potential and technological advancements.
Further, Anyscale asserts a commendable position within machine learning performance metrics, excelling particularly in the time-to- first-token metric, according to the LLMPerf Leaderboard, a public and open-source dashboard showcasing the performance of leading LLM inference providers in the market.
Anyscale achieved a leading time of just 0.21 seconds, outpacing all competitors in the race to deliver the initial output. While Groq dominates in the output-tokens-throughput with an impressive 185 tokens per second, Anyscale stands out as the top performer among the rest, demonstrating significant throughput capabilities at 66 tokens per second. This dual achievement not only showcases Anyscale’s rapid response in starting processes but also its robust throughput performance, marking it as a balanced and agile contender in the field. Anyscale’s impressive figures in both initiating and processing tasks affirm its efficient platform, catering to clients who value swift commencement of tasks without sacrificing processing speed.
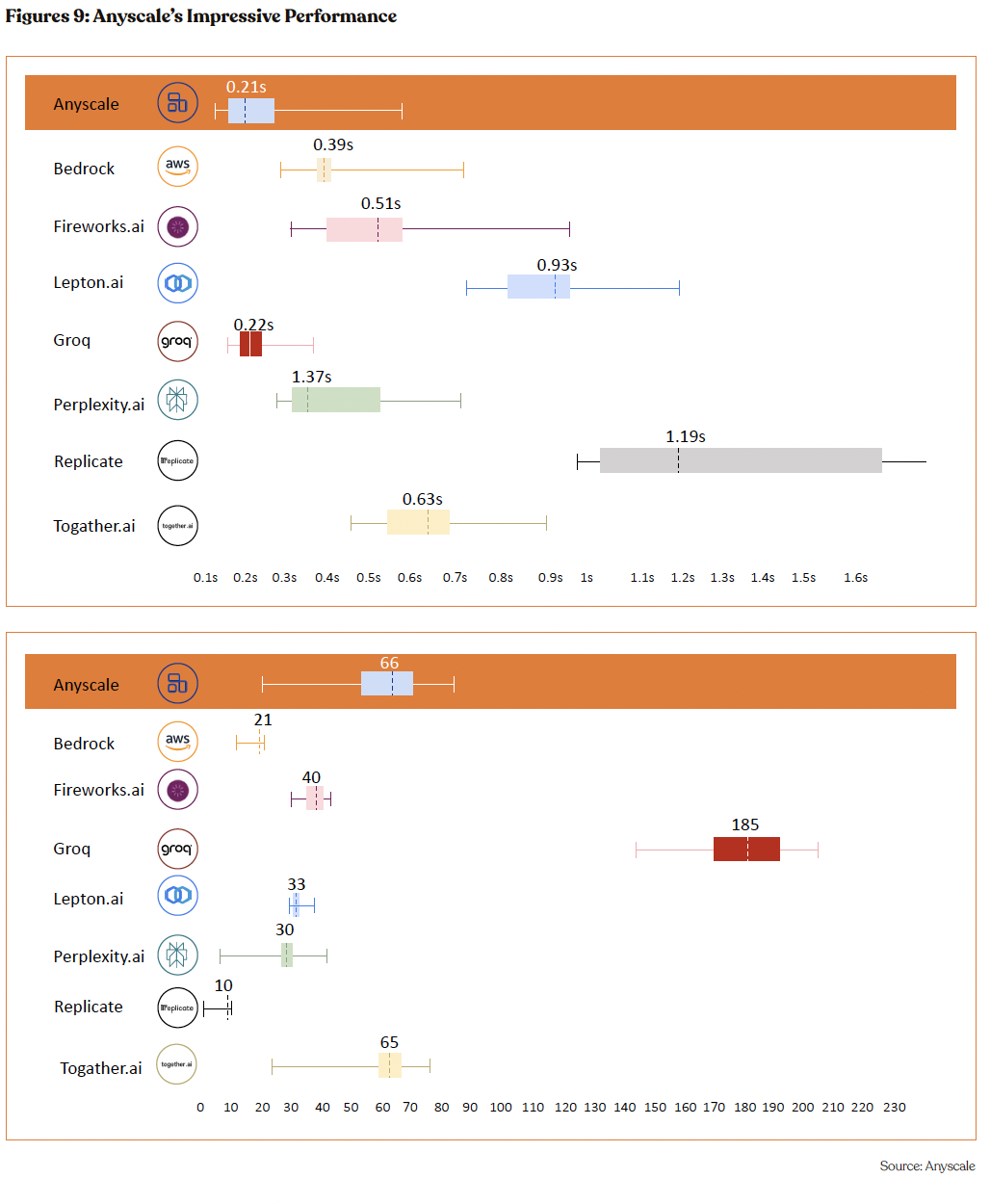
Cost-Effectiveness Vis-à-vis Proprietary AI Systems: Anyscale positions itself as a cost-effective leader in the AI and distributed computing market, offering an appealing financial advantage over proprietary AI systems.
It provides access to LLMs such as Llama 2 at a remarkably low rate of $1 per million tokens, marking a significant (50%) cost reduction when compared to other proprietary solutions. Such pricing not only enhances Anyscale’s market competitiveness but also broadens the accessibility of advanced LLMs to a wider developer community.
Moreover, Anyscale extends its cost advantages to the development of Retrieval-Augmented Generation (RAG) applications, especially in data embeddings. These processes are essential for efficient search and retrieval in vector databases but are typically resource-intensive, involving both CPU and GPU computing. Anyscale allows users to produce these embeddings at only 10% of the cost associated with other major platforms, enabling cost-effective deployment of complex AI functionalities.
The CloudSort Benchmark serves as a testament to Ray’s cost-efficiency at scale. In 2022, Ray established a new world record on AWS for sorting 100 Terabytes of data at a cost of $0.97 per TB, surpassing the previous record held by Apache Spark on Alibaba Cloud, which stood at $1.44 per TB in 2016. This achievement not only demonstrates a 33% cost reduction from the former state-of-the-art but also st saving when considering the downward trend in hardware expenses. These figures solidify Ray’s market position as a cost-effective solution for data-intensive operations.
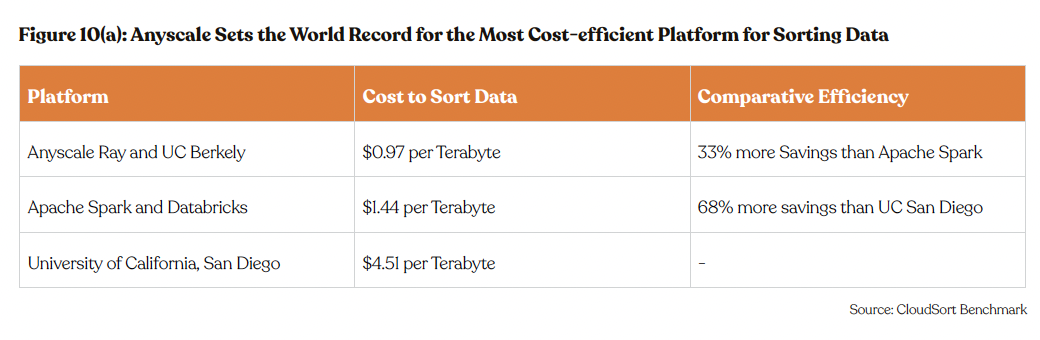
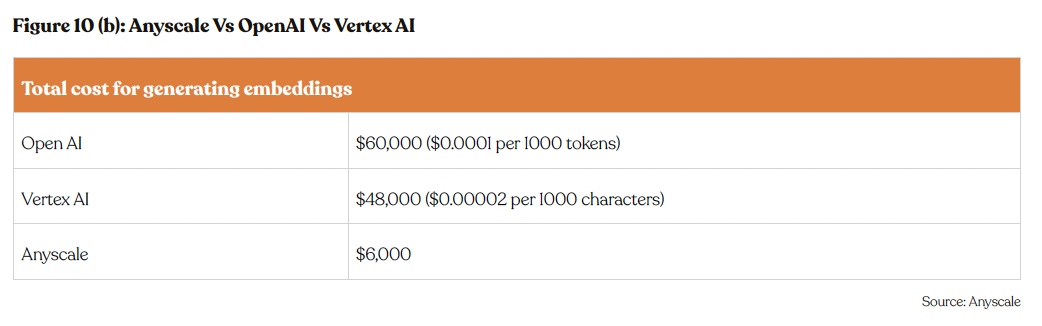
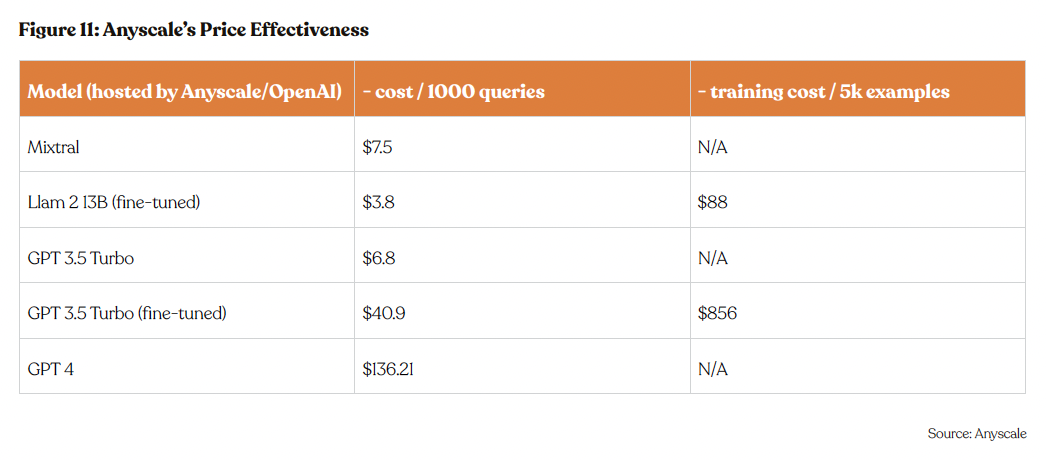
The fine-tuning capability of Anyscale Endpoints is a pivotal benefit for clients seeking to maximize their AI potential without incurring exorbitant expenses. The fine-tuned Llama-2-7B model via Anyscale Endpoints achieves an 86% task-specific performance, surpassing the 78% performance of GPT-4. This improvement is achieved at a fraction of the cost — 1/300th the cost of GPT-4, to be precise. This metric is a testament to Anyscale Endpoints’ ability to deliver enhanced AI performance while also driving down costs, making it an attractive solution for businesses looking to leverage high-performing AI models economically.
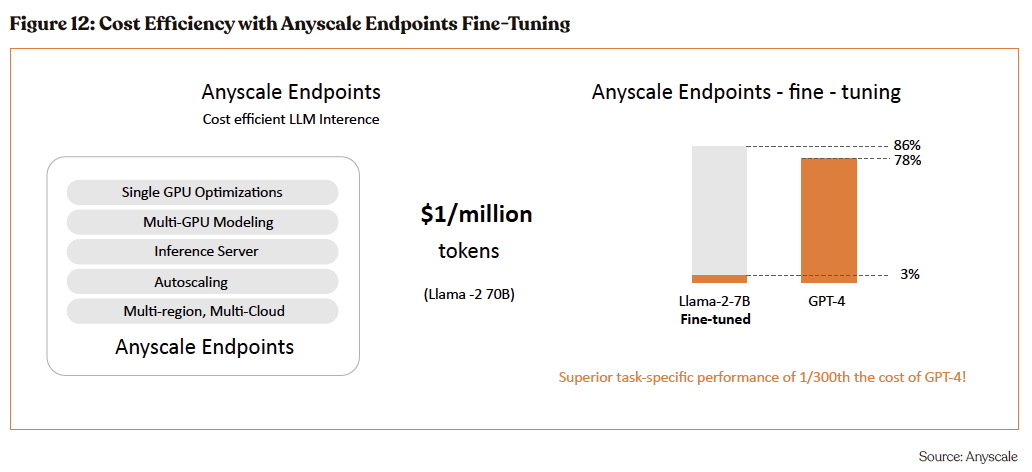
Ray boasts remarkable efficiency improvements, such as accelerating processes for Instacart by 12 times and enhancing speed for Clari by 5 times. Moreover, it offers significant cost reductions, cutting expenses for Samsara by 50%, Amazon by 10 times, Pinterest by 40%, and DoorDash by 30%. These impressive metrics not only highlight Ray’s compelling value proposition but also demonstrate its capacity to deliver substantial economic advantages across a variety of industries, from e-commerce and data analytics to social media and food delivery.
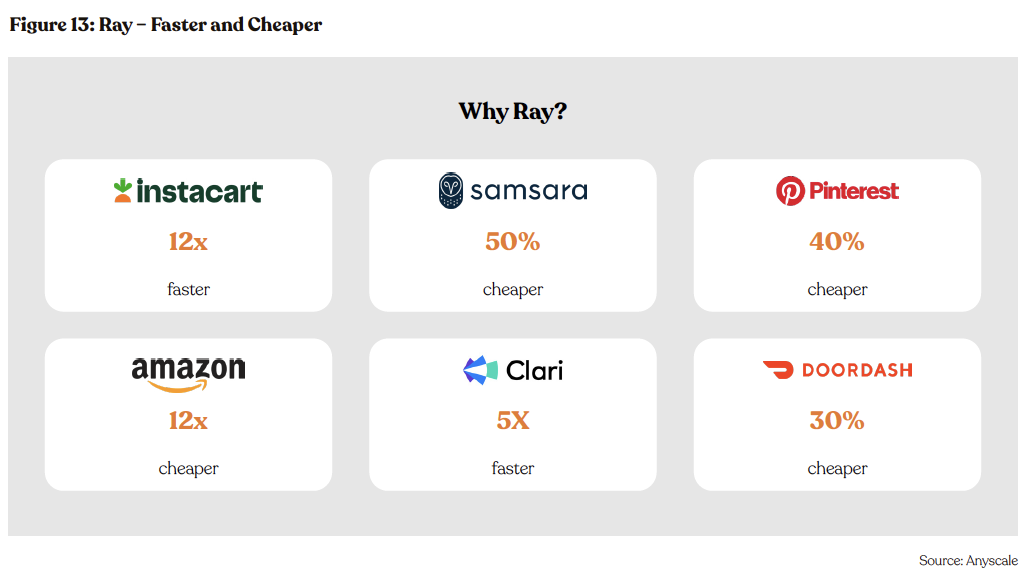
Growing Open-Source Model Adoption to Support Growth: LLMs are becoming increasingly commoditized with over 30 LLMs now in the arena including open-source models. Market dynamics have dramatically shifted, as evidenced by an Arize survey indicating OpenAI’s market share in LLM adoption plummeting from 83% in April 2023 to just 13% six months later, amidst rising alternatives. This market realignment underscores the burgeoning competition, and shifts focus beyond model capabilities to the robustness of the infrastructure that supports them.

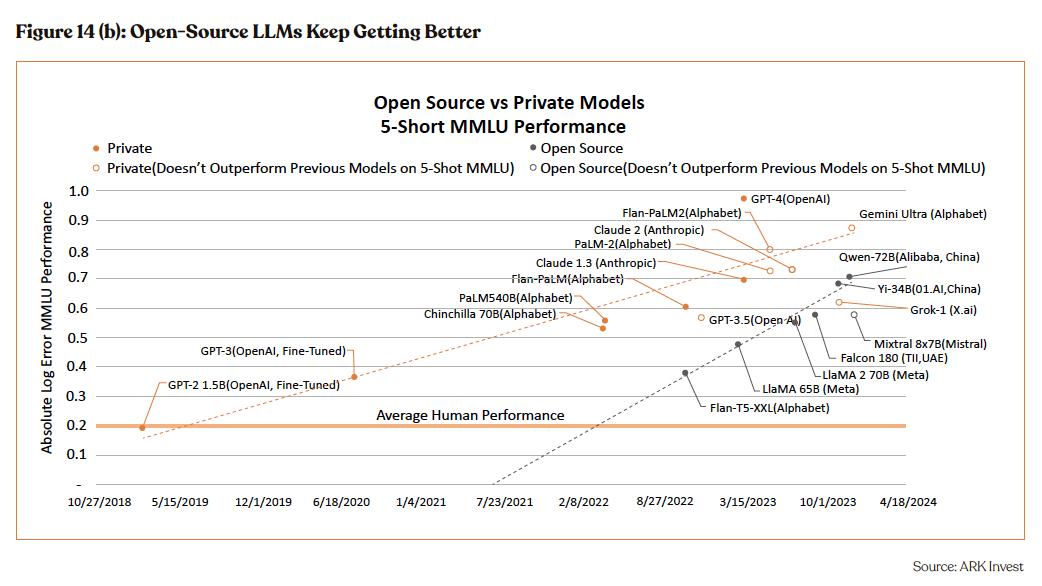
High Scalability Across Industries
Anyscale’s solutions transcend specific sectors, demonstrating versatile applications across diverse industries, encompassing finance, healthcare, automotive, and more. This broad scalability underscores the widespread demand for efficient distributed computing solu- tions, presenting a substantial market opportunity for Anyscale.
Ray, a key component of Anyscale’s offerings, has been embraced by a spectrum of organizations, including notable enterprises such as Goldman Sachs, Dow Chemical, and Linkedin, as well as major cloud platforms like AWS and Azure, along with innovative startups like Weights & Biases, Pathmind, and SigOpt. Noteworthy open-source data and machine learning projects, including Hugging Face, Horovod, and Seldon, leveraging Ray for distributed computing, further solidifying its industry - wide relevance.
In a recent development, Uber utilized Ray to enhance elastic capabilities within Horovod, their widely us ted training framework, illustrating the practical and impactful implementation of Anyscale’s solutions in real-world scenarios.
"We believe that Ray will continue to play an increasingly important role in bringing much- needed common infrastructure and standardization to the production machine learning ecosystem, both within Uber and the industry at large." - Uber Leadership
Anyscale has become the preferred choice for a diverse range of companies, spanning startups to public players, aiming to expedite and optimize the development and deployment of their AI products. Catering to industry leaders like OpenAI, Cohere, Uber, Meta, and IBM, among others, Anyscale’s platform empowers these companies to efficiently develop, run, and scale AI workloads, facilitating a rapid and cost-effective route to market for their AI products.
Anyscale’s capabilities span three pivotal categories. Firstly, it empowers AI-powered products, exemplified by Uber utilizing Anyscale to optimize costs, travel times, and estimated time to destination for riders. Secondly, it supports AI assistants powering chatbots, like Airbnb’s virtual travel agent. Lastly, it facilitates AI automation, with an instance where a customer efficiently inspects extensive footage of underground pipes for signs of wear and tear to pre-emptively address maintenance needs.
Notably, Anyscale’s solutions are highly adaptable, offering customization for on-site, cloud, or private data center deployment, optimizing the platform for practitioners. By addressing intricate challenges at the developer level, Anyscale provides those involved in mission- critical AI tasks with increased opportunities to deliver exceptional products at the application level, consequently enhancing overall business value.
Like the transformative impact of Amazon’s bookstore and the advent of the internet, the widespread benefits of AI are imminent. cking AI’s full potential necessitates a robust AI infrastructure, a challenge that Anyscale confronts head-on, positioning
Efficient Horizontal Scalability Offers Competitive Edge
The demand for distributed computing solutions is growing, driven by the increasing need for processing large datasets, real-time data analytics, and machine learning models. Anyscale’s position in this high-growth market, with its user-friendly platform for building and scaling applications, positions it well to capture a significant share of this expanding market.
In the development of a distributed application, the necessity for resources such as CPUs or GPUs for tasks is inherent. Optimal performance is achieved when these resources are seamlessly available without the need for pre-planning or post-utilization disposal. Ray Clusters offer a solution to this challenge by proficiently handling the scheduling of all required resources for a Ray application. A key highlight is the automated resource management facilitate by the Ray Autoscaler, dynamically responding to the application’s resource demands, ensuring efficiency without manual intervention.
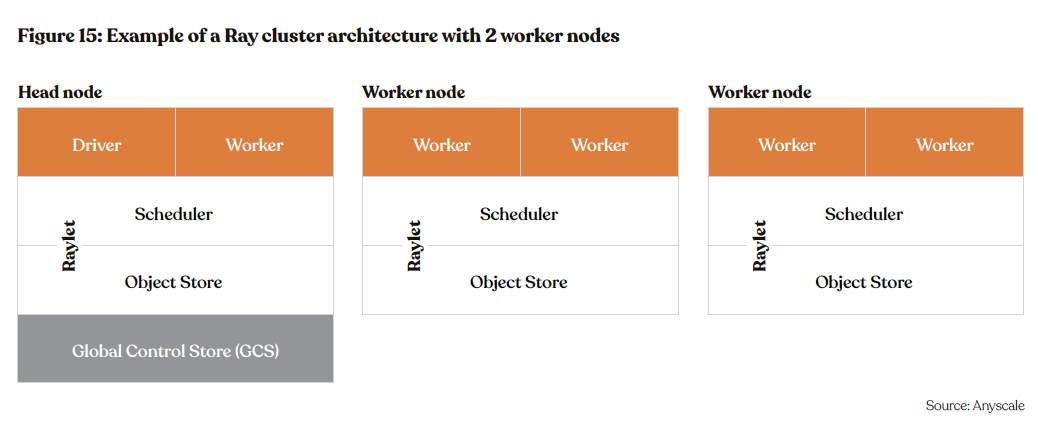
Each node type, comprising both the head node and workers, presents a resource collection (e.g., 4 CPUs per node), specified in the defi- nition or automatically identified. Armed with a comprehensive understanding of the resources offered by each node and guided by ap- plication demands, the Ray Autoscaler adeptly assesses the need for additional nodes. Conversely, in instances where available resources surpass the application’s requirements, resulting in idle nodes, the Autoscaler efficiently shuts down such nodes.
The Ray Cluster serves as a dynamic mechanism for horizontally scaling distributed applications. Leveraging Ray, scaling existing appli- cations to a cluster is streamlined with minimal code modifications and devoid of intricate infrastructure complexities. This streamlined approach empowers efficient processing of extensive datasets within minutes, a significant improvement compared to traditional methods requiring hours for similar tasks.
Competitive Edge Competent and Experienced Leadership Team
Anyscale’s impressive leadership team is a vital asset that positions the company for continued growth, innovation, and effective solutions within the evolving LLM orchestration landscape.
Ion Stoica, Co-founder and Executive Chairman: Ion Stoica, the executive chairman of Anyscale, brings a wealth of expertise to the intersection of academia and industry. Serving as a professor at the University of California, Berkeley, he has authored over 100 peer-re- viewed papers in areas such as cloud computing, networking, distributed systems, and big data. Before assuming his role at Anyscale, Ion held the position of Co-founder and CEO at Databricks, a California-based data and AI startup. Preceding his tenure at Databricks, he served as the CTO at Conviva Inc., a California-based video streaming platform. Ion’s distinguished background, marked by leadership roles in tech startups and a comprehensive understanding of data and AI, establishes a robust technological foundation for Anyscale. His academic journey culminated in a Ph.D. from Carnegie Mellon University.
Robert Nishihara, Co-founder and the CEO: Robert Nishihara, the CEO of Anyscale, has deep experience in the artificial intelligence and machine learning space through internships in organizations such as Microsoft and Facebook. Robert obtained his bachelor’s degree in mathematics from Harvard University and a master’s degree in computer science from the University of California, Berkeley.
Philipp Moritz, Co-founder and the CTO: Philipp Moritz has a master’s degree in mathematics from the University of Cambridge and a PhD from the University of California, Berkeley.
Tricia Fu, Product Manager: Tricia Fu has a bachelor’s degree in electrical engineering and computer science from the University of California. Before joining Anyscale, she held leadership positions in technology companies such as Google and LinkedIn.
Customer Testimonials Cement Credibility
The platform helped Wildlife Studios, a mobile gaming company, to significantly speed up time-to-market on new products. Spotify uses Anyscale’s Ray to power machine learning applications like recommending personalized content, search ranking, content discovery, etc.
My team at Afresh trains large time-series forecasters with a massive hyperparameter space. We googled Pytorch hyperparameter tuning and found Ray Lightning. It took me 20 minutes to integrate into my code, and it worked beautifully. I was honestly shocked. - Philip Cerles, Senior Machine Learning Engineer
Anyscale helped Doordash, a California-based online food ordering platform, with 10-20X gains in performance of their platform.
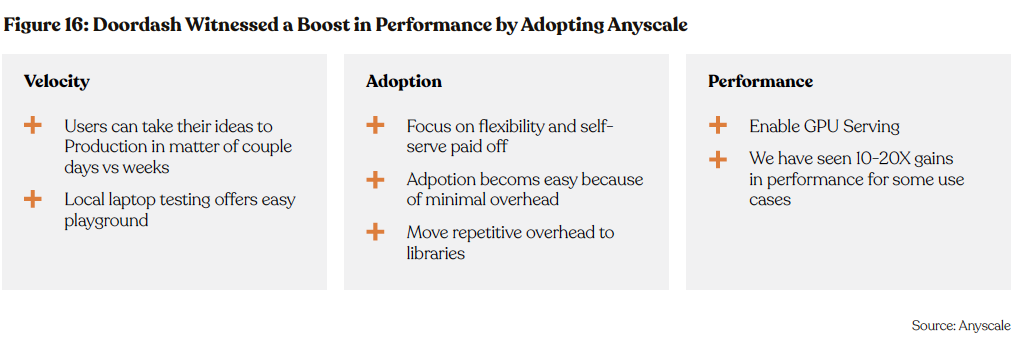
Anyscale also helped Ant Group, a Chinese digital payments company, that implemented scalable Ray Serving architecture atop Ray in 2022, deploying 240,000 cores for model serving, scaling by 3.5x from 2021.
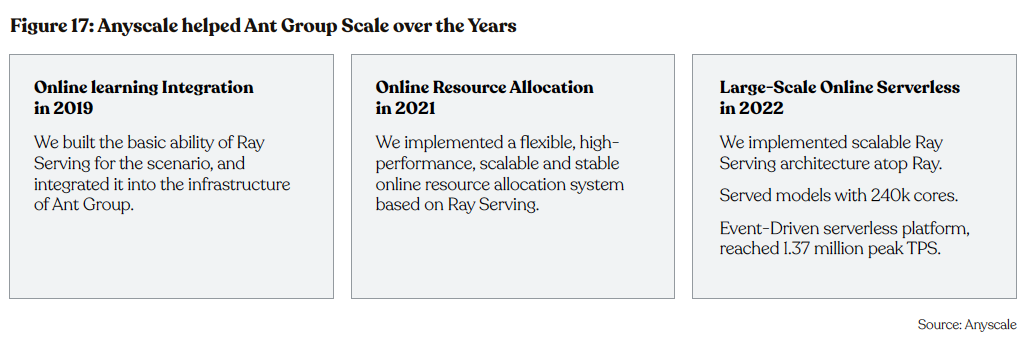
Through the integration of extensive deep learning capabilities within a Ray heterogeneous cluster (CPU + GPU), the Uber team achieved a remarkable 50% reduction in ML compute costs for large-scale deep-learning training. The Uber internal Autotune service, now leveraging Ray Tune, witnessed an impressive up to 4x acceleration in hyper parameter tuning processes.
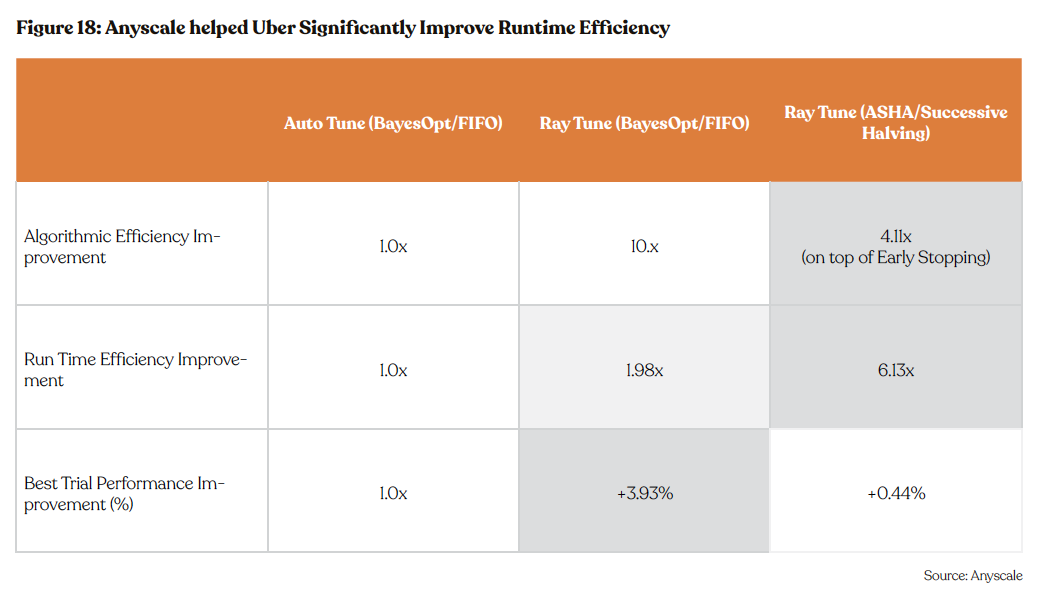
Key Investment Concerns
Reluctance to Change to Hinder Adoption
If an organization has institutional knowledge of the Spark API, a longstanding peer platform since 2014, and the workloads are data-cen- tric and more around ETL (extract, transform, load)/pre-processing, it is unlikely that the organization will switch to Ray, a relatively recent contender. However, the clear superiority of Ray over Spark coupled with a broader use case in AI, will propel the adoption in the foresee- able future.
Historically, programmers exhibit a notable reluctance towards change, particularly in the realms of coding style, language, and tools. This resistance stems from a substantial investment of time and effort in mastering current practices, with concerns over potential declines in code efficiency and readability. This resistance is grounded in the fear of the unknown, as change introduces disruptions that are chal- lenging to foresee and may impact workflow. Additionally, attachment to the existing status quo and comfort with established methods contribute to programmers’ hesitancy to embrace new approaches. Furthermore, apprehensions about making costly mistakes in the face of inherent risks associated with change add another layer to their resistance.
For reference, Agile, a group of software development methodologies launched in 2001, provides 60% more revenue growth than other methodologies. Evne though, 34% organizations face resistance to agile adoption. The reasons for resistance are presented in the chart below.
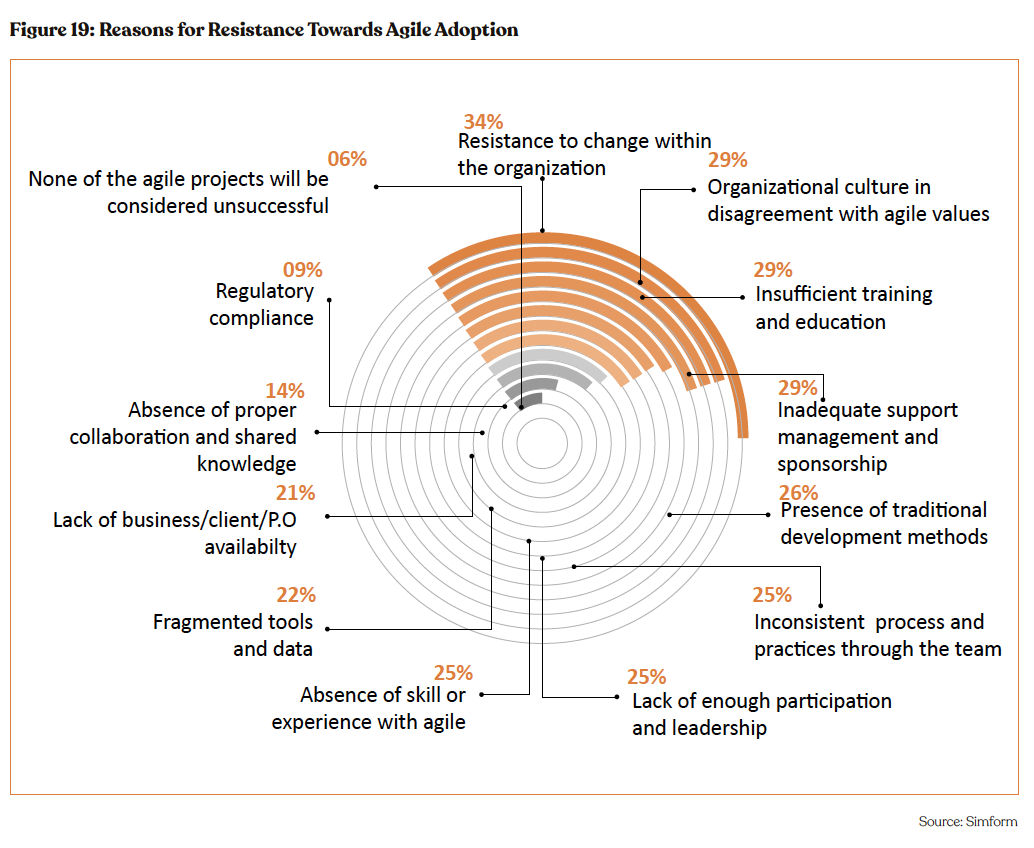
Anyscale's Open-Source Focus - a Double Edge Sword
Anyscale’s growth strategy is intricately linked with the accelerating adoption of open-source models, a trend that is vital for the company’s expansion. While the open-source movement holds the promise of democratizing AI development and fostering innovation, its trajectory faces potential headwinds that could impact Anyscale.
Despite the growing appetite for transparent and accessible AI, significant barriers such as the scarcity of public training data and the requisite compute infrastructure could impede the momentum of open-source AI projects. Firstly, the lack of publicly available training data restricts developers’ ability to modify and retrain open-source models effectively. While altering a model’s source code might be straightforward, the absence of original training datasets hinders any substantive retraining or enhancements, limiting the adaptability and evolution of open-source AI solutions. Moreover, without transparency in training data, the operations of an open-source model can remain opaque, undermining the very premise of open-source AI to offer full transparency and trustworthiness.
Secondly, the compute resources necessary for training sophisticated AI models represent a significant financial hurdle. The substantial costs associated with these resources pose a challenge, particularly for smaller companies. While some companies, like Mistral AI, have navigated these obstacles through raising funds, this strategy is not universal y for smaller projects or firms that are hesitant to allocate substantial funds towards training open-source AI models.
Dedicated Chips for AI Inference to Intensify Competition
Groq, a semiconductor startup focused on making chips for LLMs, has gained notable attention for its impressive demonstrations featuring the Mistral Mixtral 8x7b, an open-source model. Achieving a remarkable 4x throughput compared to competitors, Groq established itself apart by offering pricing that is less than one-third of Mistral’s rates. Central to this groundbreaking innovation is the GroqCard Accelerator, available at $19,948, priced close to NVIDIA, delivering unparalleled performance with up to 750 TOPs (INT8) and 188 TFLOPs (FP16 @900 MHz). Boasting 230 MB SRAM per chip and up to 80 TB/s on-die memory bandwidth, it outperforms traditional CPU and GPU setups, particularly excelling in LLM tasks. This remarkable performance leap is attributed to the LPU’s capacity to significantly reduce computation time per word and mitigate external memory bottlenecks, enabling accelerated text sequence generation. Other chip companies such as NVIDIA, AMD, Cerebras, are poised to come up with such LLM-dedicated chips posing strong competition to Anyscale’s offerings.
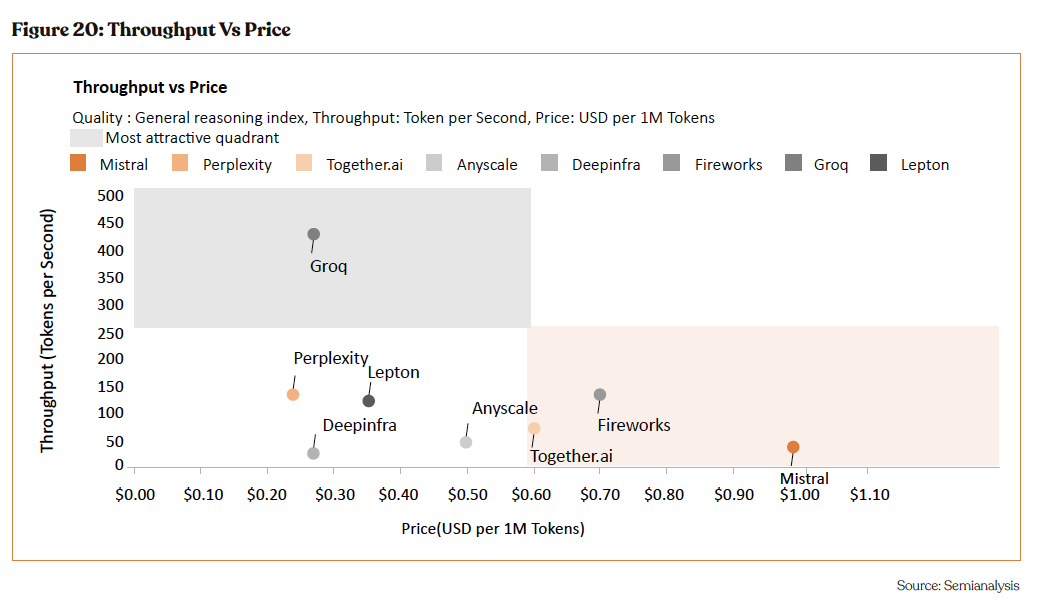
However, as evidenced by the case of NVIDIA, which struggles to satisfy only 50% of chip demand, meeting abrupt surges in demand
poses a formidable challenge for hardware companies. Therefore, Groq and other similar companies are likely to encounter si lar challenges, creating an opportunity for Anyscale, an asset-light startup, to seize a substantial share in this rapidly expanding market.
Industry Overview
Enterprises grapple with the imperative of constructing scalable machine-learning solutions to handle burgeoning data volumes. The scalability of machine learning workloads is influenced by various factors, including the selection of ML and data processing frameworks, access to scalable compute resources, deployment flexibility, and the availability of skilled personnel. The CEO of Anyscale highlights that the compute requirements for AI applications are doubling every 3.5 months. Anyscale’s flagship product, Ray, is position revolutionize a lucrative $13 trillion market opportunity in AI, according to David George, General Partner at Andreessen Horowitz.
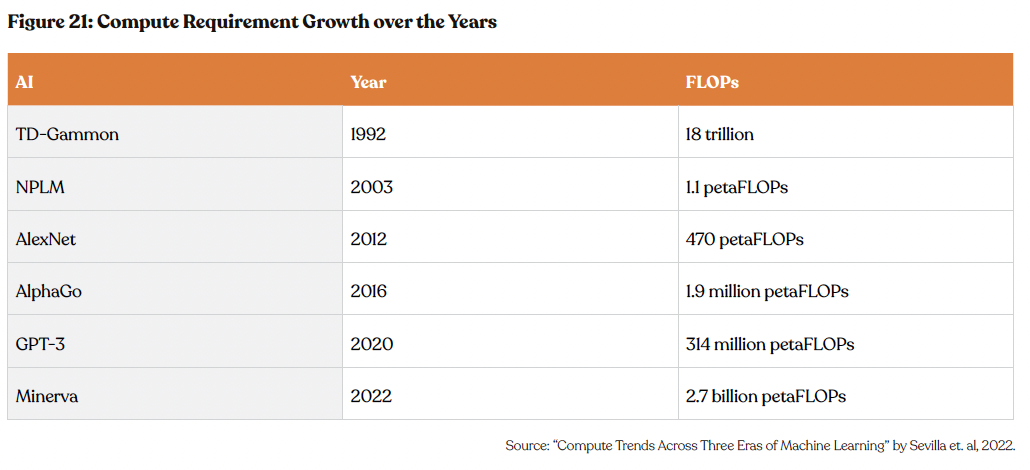
LLM orchestration is the strategic administration and regulation of large language models to enhance their performance and efficacy. This encompasses formulating impactful prompts, chaining outputs from multiple LLMs for results, optimizing resource allocation, and vigilant performance monitoring through metric tracking for issue resolution.

LLM orchestration can be pictured as a strategic planner akin to an aircraft dispatcher, where operational efficiency directly correlates with adept task handling—route planning, weather checks, precise communication, and coordination with external entities. Similarly, LLM orchestration strategically guides how your application interacts with large language models, ensuring a coherent conversation. Skillfully executed, it results in accurate information sharing and seamless operations.
To fully harness LLM capabilities, a well-designed orchestration framework, is imperative. Serving as the central nexus, this framework harmoniously blends diverse AI technologies within a broader AI network, unlocking their synergistic potential. Implementation demands applications like GenAI and backend systems such as enterprise resource planning databases.
LLM orchestration stands as a crucial toolkit for unlocking the expansive potential of large language models. By offering a structured and efficient management approach, it empowers developers to construct robust, scalable, and reliable applications that leverage the trans- formative power of this cutting-edge technology.
As LLMs advance and evolve in sophistication, the role of LLM orchestration will expand. Developers will increasingly rely on these frame- works to navigate LLM complexity, optimize performance, and seamlessly integrate them into applications. The future of LLM orchestra- tion is promising, set to play a pivotal role in shaping the AI-powered application landscape for years to come.
The large and growing total addressable market around LLM orchestration, underscores the huge scope of this technology in the coming years. For instance, the global generative AI market is projected to grow from $11.3 billion in 2023 to reach $76.8 billion in 2030, at a CAGR of of 31.5%, per MarketsandMarkets.
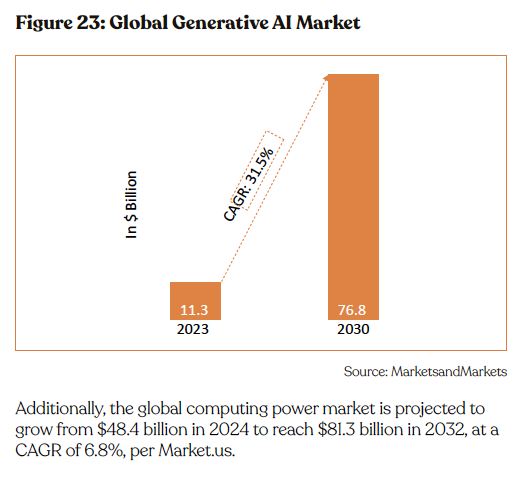
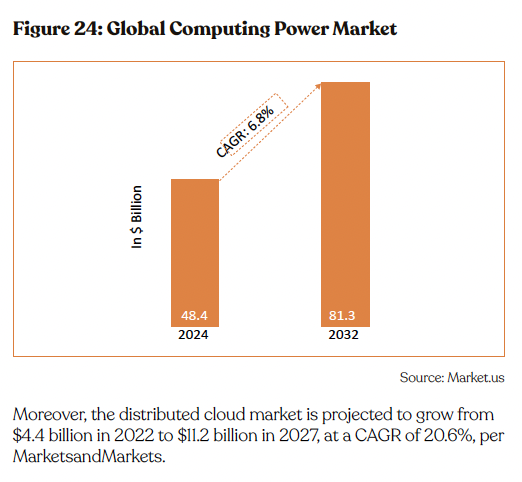

Financials

Anyscale demonstrated robust financial performance in 2021, achieving an estimated annual revenue of $45.1 million, equating to an impressive $157,867 per employee according to Startup Talky. Additionally, the company experienced substantial growth, markable 101% increase in employee count and a monthly web visit surge to 55,699, boasting an impressive growth rate of 18.62%.
Funding Rounds and Valuation
Anyscale has secured $259.6 million in funding across four funding rounds. Anyscale’s approach to streamlining the scale of machine- learning workloads has resonated with both customers and investors, leading to significant investor interest since 2021. Notably, the company raised $100 million in 2021. The company’s ability to attract investment has continued to grow, with its latest funding round – a
$99 million Series C-II in August 2022 with Addition and Intel Capital as lead investors and participation from Foundation Capital. The Series C round valued the company at $1 billion, a 447% markup from its valuation of $182.85 million post-series B in October 2020.
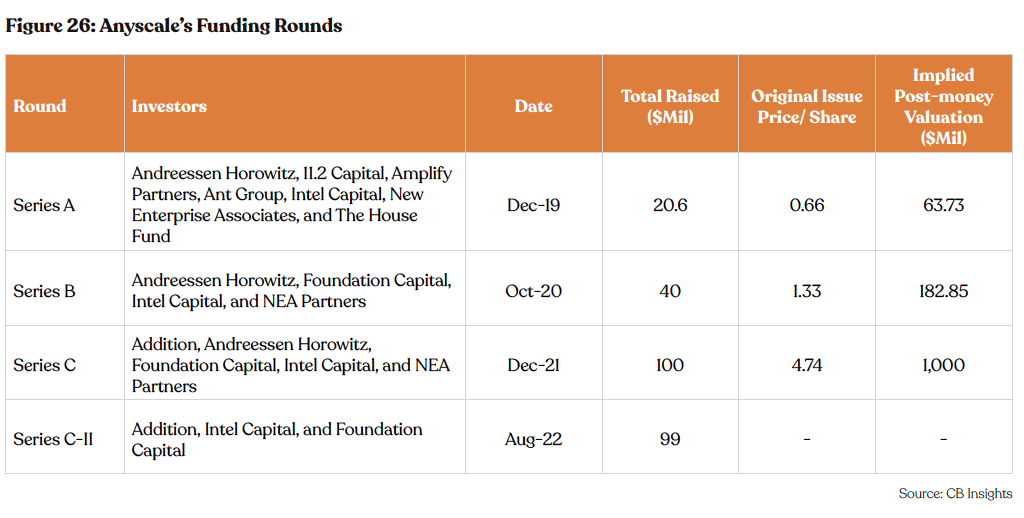
Private Market Valuation
Anyscale raised its Series C round in December 2021 at a valuation of $1 billion. Since its last funding, the generative AI sector has undergone transformative growth, suggesting that Anyscale’s market value could climb further.
The valuation benchmarks set by AI industry peers are indicative of a robust appetite for AI-driven firms, with increases ranging from 58% to 550%, according an MVR analysis of select mid-stage AI infrastructure start-ups. These figures suggest that the market is increasingly recognizing the substantial future value these companies are likely to deliver.
In 2023, early-stage AI companies commanded substantially higher valuations compared to their non-AI counterparts. This trend per- sists into the mid-stages, where AI startups raising Series B rounds achieved valuations exceeding 1.5 times those of non-AI peers, per CB Insights. The versatile applications of generative AI have attracted investors throughout the value chain of the burgeoning AI industry.
Taking into account the industry’s trajectory and Anyscale’s unique value preposition, technological strategic client relationships, we can extrapolate a continued, if not accelerated, growth trajectory for Anyscale’s valuation.
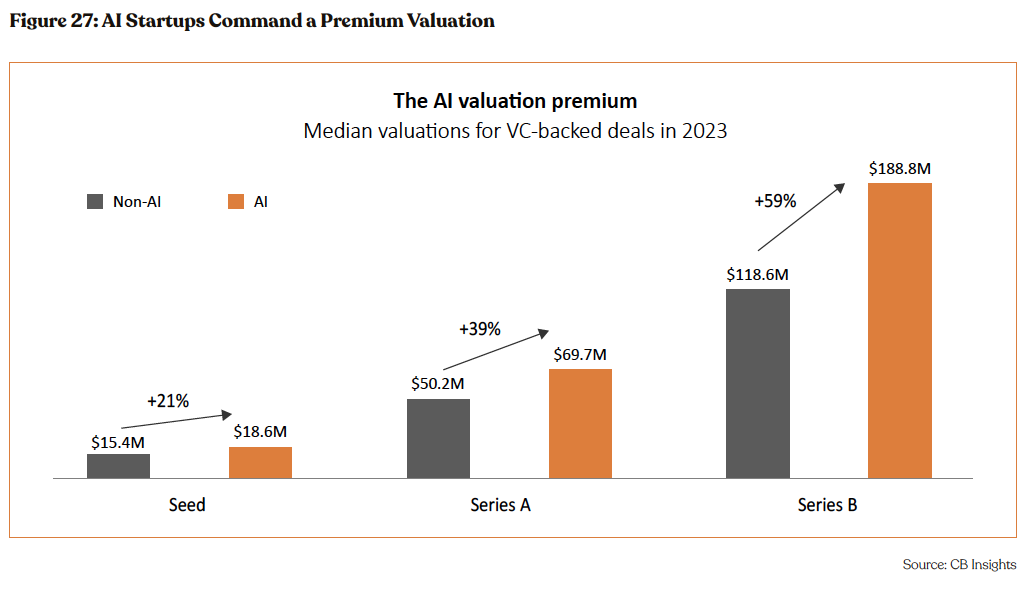
Our analysis, examining mid-stage (B, C, or D rounds) valuation markups among various AI infrastructure layer startups, reveals a median markup of 151%. Extrapolating this markup to 151%. Extrapolating this markup to Anyscales' Series C valuation, we project a current valuation of $2.5 billion in the event of another funding round, IPO, or acquisition.
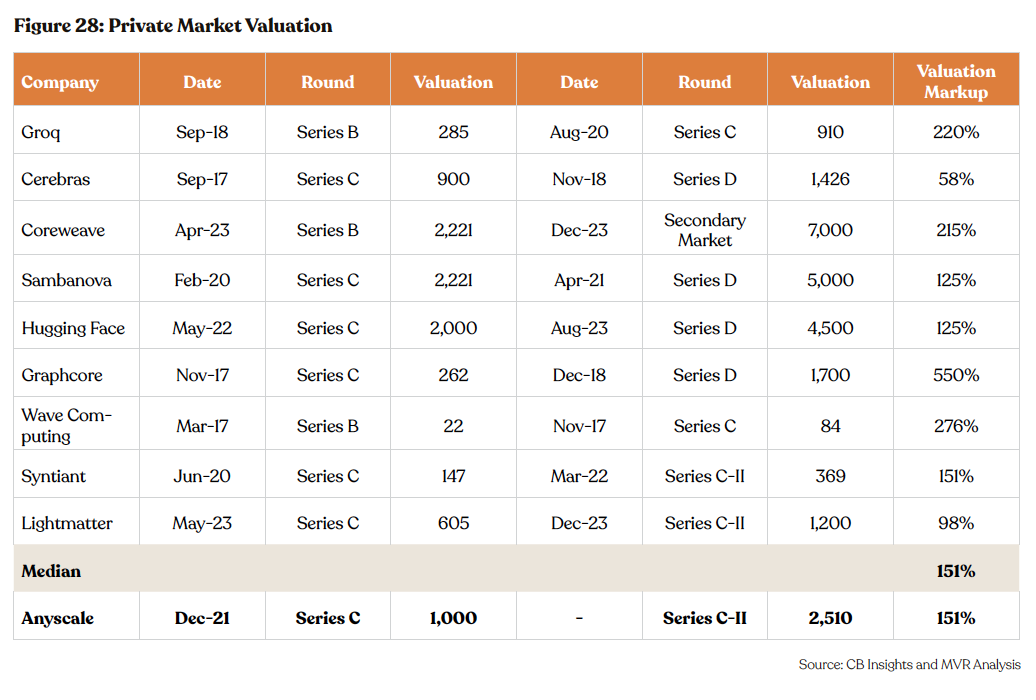
Comparative Public Multiples
The following table shows the multiple of public companies operating in enterprise software and enterprise AI space. The public multiples provide a useful reference point for Anyscale’s future valuation. Given the strong track company in LLM orchestration, it is reasonable to expect Anyscale to command a premium over its public peer multiples.
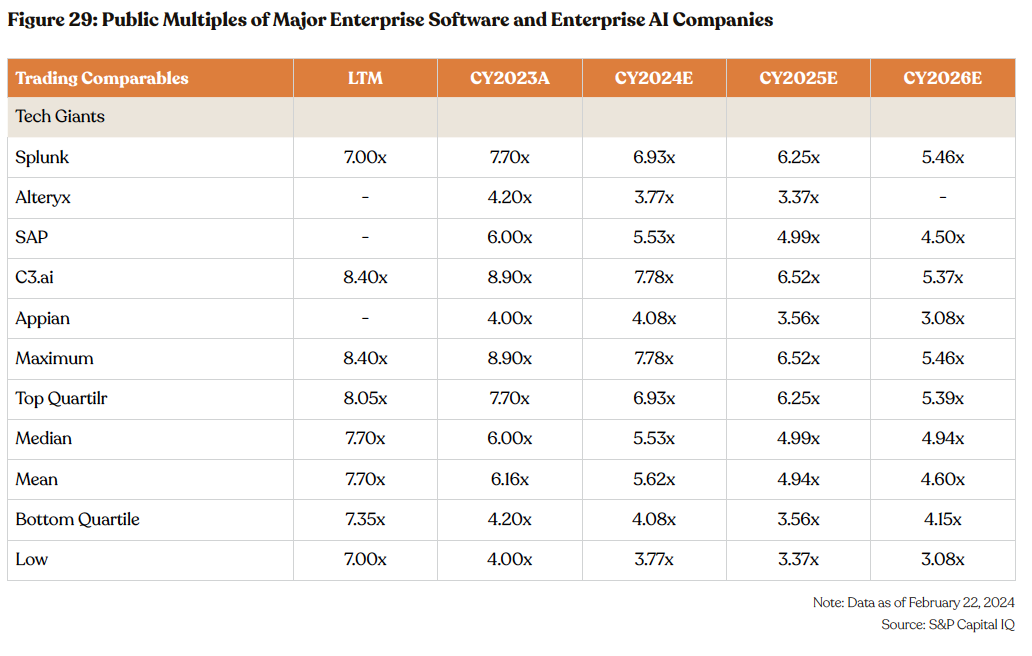
About the Analyst
Santosh Rao
Santosh Rao has over 25 years of experience in equity research with a primary focus on the technology and telecom sectors. He started his equity research career at Prudential Securities and later moved to Dresdner Kleinwort Wasserstein, Gleacher & Co, and Evercore Partners, where he followed Telecom and Data Services. Prior to joining Manhattan Venture Partners, he was the Managing Director and Head of Research at Greencrest Capital, focusing on private market TMT research. Santosh has an undergraduate degree in Accounting and Economics, and an MBA in Finance from Rutgers Graduate Business School. While at Gleacher & Co he was ranked leading telecom equipment analyst by Starmine/Financial
Times
Disclaimer
I, Santosh Rao, Head of Research, certify that the views expressed in this report accurately reflect my personal views about the subject, securities, instruments, or issuers, and that no part of my compensation was, is, or will be directly or indirectly related to the specific views or recommendations contained herein.
Manhattan Venture Research is a wholly-owned subsidiary of Manhattan Venture Holdings LLC (“MVP”). MVP may currently and/or seek to do business with companies covered in its research report. As a result, investors should be aware that the firm may have a conflict of interest that could affect the objectivity of this report. Investors should consider this report as only a single factor in making their investment decision. This document does not contain all the information needed to make an investment decision, including but not limited to, the risks and costs.
Additional information is available upon request. Information has been obtained from sources believed to be reliable but Manhattan Venture Research or its affiliates and/or subsidiaries do not warrant its completeness or accuracy. All pricing information for the securities discussed is derived from public information unless otherwise stated. Opinions and estimates constitute our judgment as of the date of this material and are subject to change without notice. Past performance is not indicative of future results. MVP does not engage in any proprietary trading or act as a market maker for securities. The user is responsible for verifying the accuracy of the data received. This material is not intended as an offer or solicitation for the purchase or sale of any financial instrument. The opinions and recommendations herein do not take into account individual client circumstances, objectives, or needs and are not intended as recommendations of particular securities, financial instruments, or strategies to particular clients. The recipient of this report must make its own independent decisions regarding any securities or financial instruments mentioned herein. Periodic updates may be provided on companies/industries based on company-specific developments or announcements, market conditions, or any other publicly available information.
Copyright 2023 Manhattan Venture Research LLC. All rights reserved. This report or any portion hereof may not be reprinted, sold, or redistributed, in whole or in part, without the written consent of Manhattan Venture Research. Research is offered through VNTR
Securities LLC.
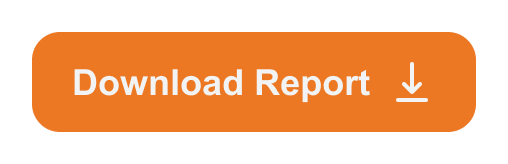
What’s a Rich Text element?
Heading 3
Heading 4
Heading 5
The rich text element allows you to create and format headings, paragraphs, blockquotes, images, and video all in one place instead of having to add and format them individually. Just double-click and easily create content.
Static and dynamic content editing
A rich text element can be used with static or dynamic content. For static content, just drop it into any page and begin editing. For dynamic content, add a rich text field to any collection and then connect a rich text element to that field in the settings panel. Voila!
How to customize formatting for each rich text
Headings, paragraphs, blockquotes, figures, images, and figure captions can all be styled after a class is added to the rich text element using the "When inside of" nested selector system.