
Venture Bytes #108: Drug Discovery Process Major Beneficiary of AI Integration

Drug Discovery Process Major Beneficiary of AI Integration
The drug discovery process is characterized by lengthy timelines, substantial expenses, and limited success rates. For instance, according to the NIH, the journey from conceptualizing a new drug to its market launch typically spans 12–15 years and incurs costs exceeding $1 billion. Contract research organizations (CROs) specialize in providing drug discovery services and are being
increasingly commissioned by pharmaceutical companies. While some pharma firms conduct in-house drug discovery, the proportion of outsourced drug discovery has risen significantly from 36.5% in 2017 to 49.3% in 2023, per Statista. The CRO market is highly consolidated, with the top 5 players experiencing stagnant revenues in 2023. In response, AI-powered drug discovery startups are emerging as attractive acquisition targets for CRO industry leaders. These acquisitions promise enhanced revenue growth and seamless integration of AI capabilities into their service portfolios.
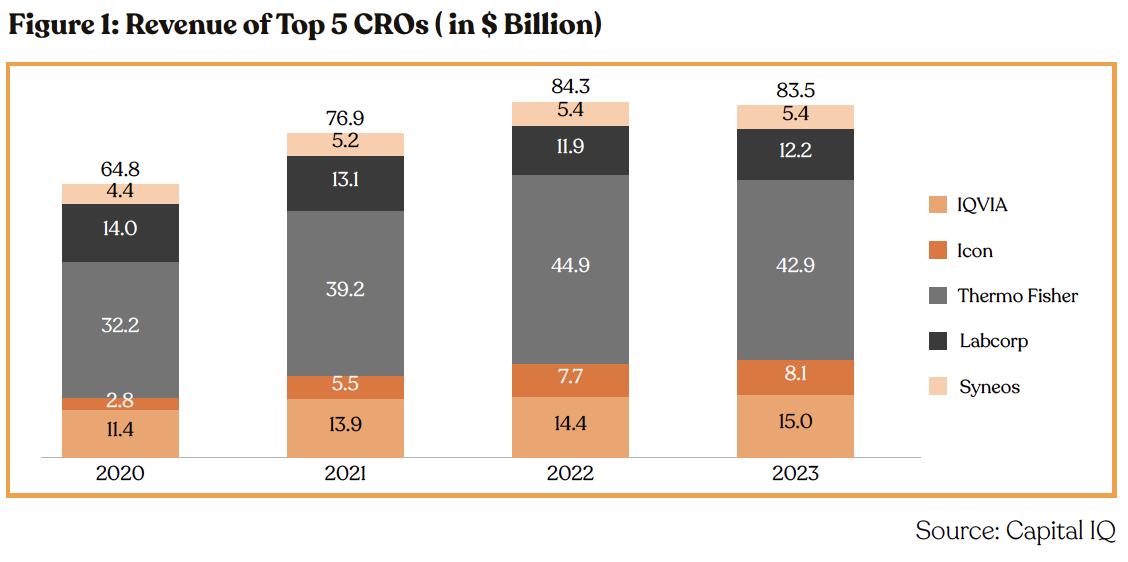
Incorporating AI technologies presents a promising avenue for enhancing drug discovery processes. AI can expedite drug discovery by forecasting molecule properties and pinpointing potential drug candidates. Machine learning algorithms sift through extensive chemical and biological datasets, uncovering patterns and correlations that facilitate the identification of new drug targets and compounds.
The rise of telehealth, huge databases in Electronic Health Records, accessibility of high computer power, and the proven tangible benefits through AI integration in various industries, are driving the adoption of AI in drug discovery. Moreover, regulatory bodies are also accepting AI integration. For instance, FDA’s Center for Drug Evaluation and Research, in partnership with the Center for Biologics Evaluation and Research and the Center for Devices and Radiological Health, has issued an initial discussion paper to engage stakeholders and explore the use of artificial intelligence and machine learning (AI/ML) in drug and biological product development. Continuous feedback is sought to advance regulatory science. Highlighting AI/ML’s significance, the FDA intends to establish a flexible, risk-based regulatory framework that encourages innovation while safeguarding patient safety.
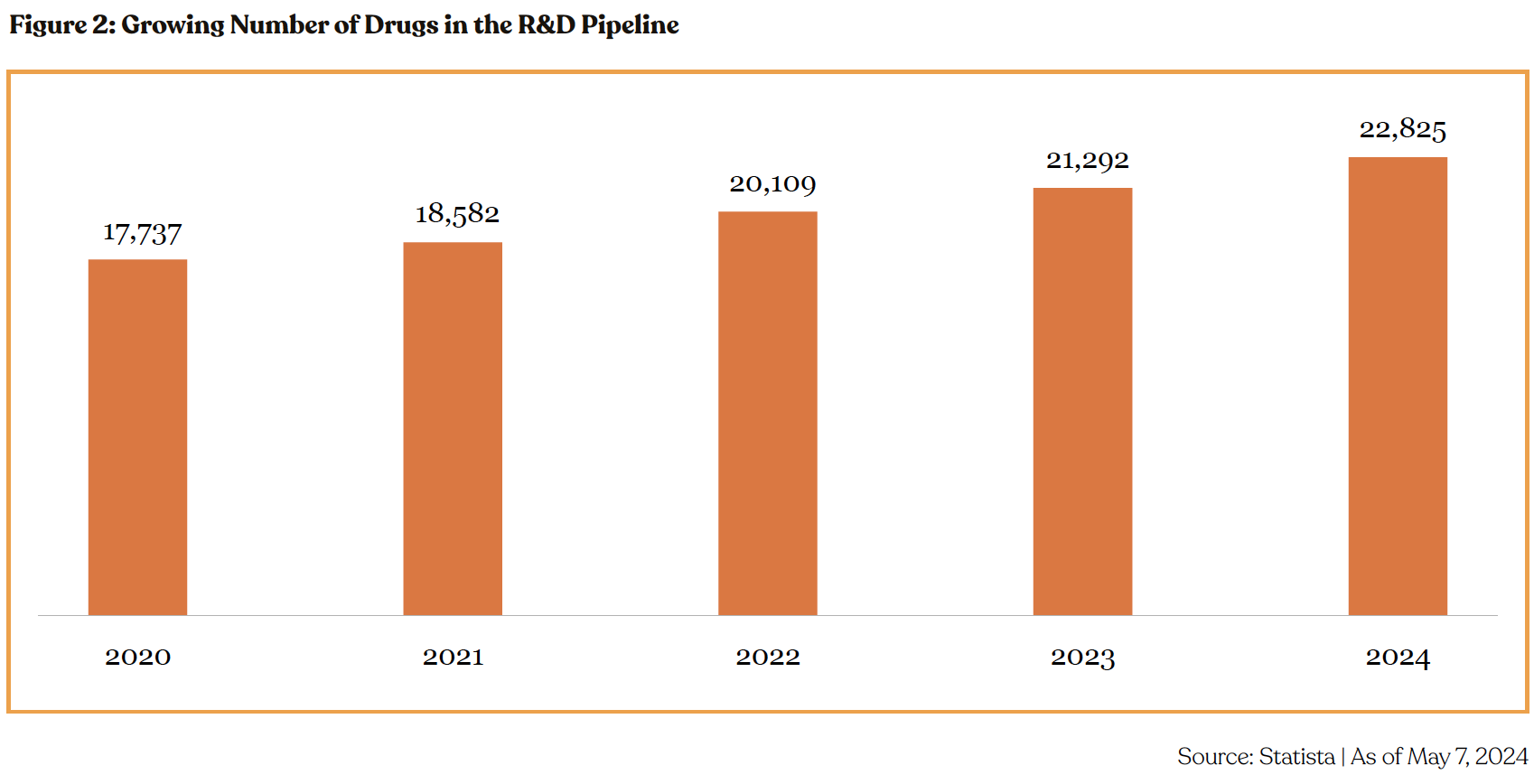
The AI in drug discovery market is projected to grow from $0.9 billion in 2023 to $4.9 billion in 2028, at a CAGR of 40.2%, per MarketsandMarkets. Startups such as Generate Biomedicines, BigHat Biosciences, Seismic Therapeutics, and Benchsci, among others, are well-equipped to capitalize on this rapidly growing market.
In addition to drug discovery, AI can significantly contribute to drug repurposing strategies, a method focused on identifying existing drugs or those with established safety profiles in humans, which can be applied to treat conditions different from their original indications. This approach expedites the delivery of effective treatments to patients awaiting medical interventions. Moreover, it offers substantial financial advantages for pharmaceutical enterprises seeking to introduce new medications to the market, in contrast to conventional drug discovery methodologies. Implementing drug repurposing can slash costs to below half a billion dollars, thereby exerting a profound influence on a company's research and development budget.
A number of promising startups are working on integrating AI in the drug discovery process. Significant among them are: Generate Biomedicines, BogHat BioSciences, Seismic Therapeutics and BenchSci.
•Massachusetts-based Generate Biomedicines integrates machine learning, medicine, and biological engineering to develop proteins with specific biophysical, biological, and therapeutic qualities. Ranked 25th on the 2024 CNBC Disruptor 50 list, the company has attracted significant investments from renowned firms including ARCH Venture Partners, Fidelity Investments, Morningside Venture Partners, NVentures, and T. Rowe Price, among others. A 29% surge in valuation in the recent funding round underscores investor faith in the startup's potential.
•California-based BigHat Biosciences stands at the intersection of drug development, machine learning, and biology. With over 10 therapeutics under development, the startup is emerging as a strong contender in AI-powered drug discovery. Strategic collaborations with pharmaceutical giants such as Merck and Abbvie affirm credibility. Marquee investors such as 8VC, Amgen Ventures, Andreessen Horowitz, and Bristol-Myers Squibb, among others, further cement the credibility.
•Massachusetts-based Seismic Therapeutics is a biotech firm revolutionizing the discovery and development of immunology therapies through machine learning. Leveraging its integrated IMPACT platform, an AI Model, the company has cultivated a robust pipeline of preclinical stage best-in-class and first-in-class biologics aimed at modulating dysregulated adaptive immunity and tackling various autoimmune diseases.
•Benches, headquartered in Canada, provides ASCEND, a GenAI platform in disease biology. ASCEND leverages comprehensive biomedical experiment data to develop a scalable AI assistant tailored for preclinical organizations. With over 4,300 prestigious academic research institutions and 16 of the top 20 pharmaceutical companies adopting BenchSci's AI solutions, it has garnered the trust of over 50,000 scientists who depend on it to inform their experiment decisions.
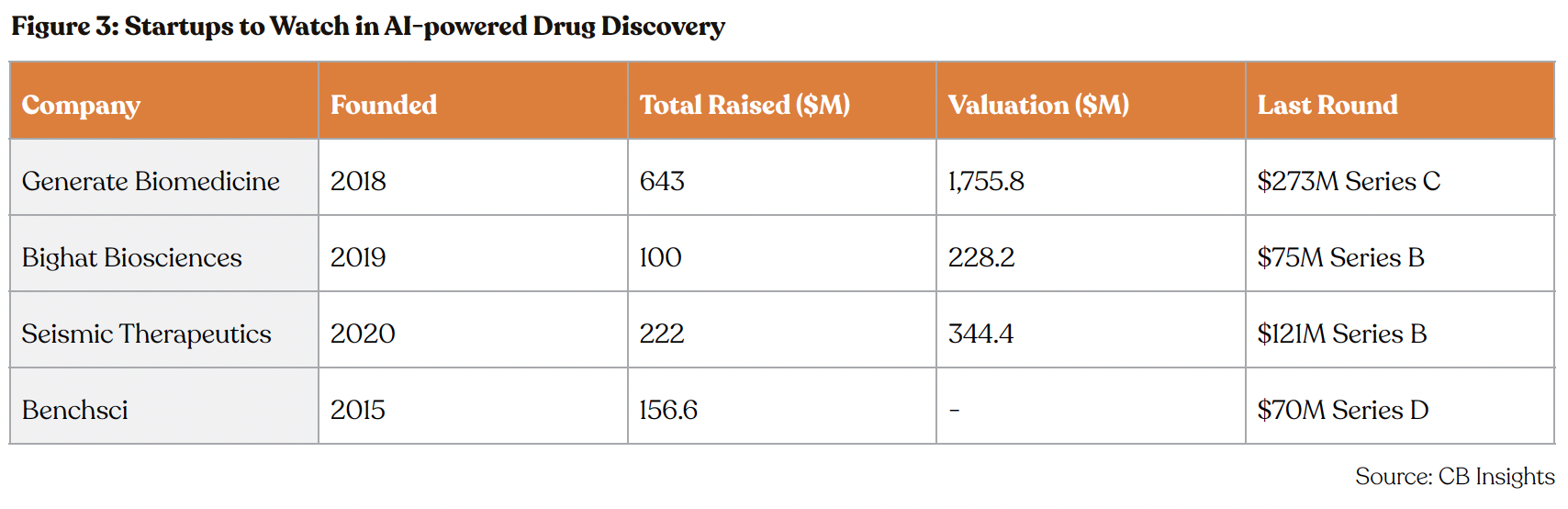
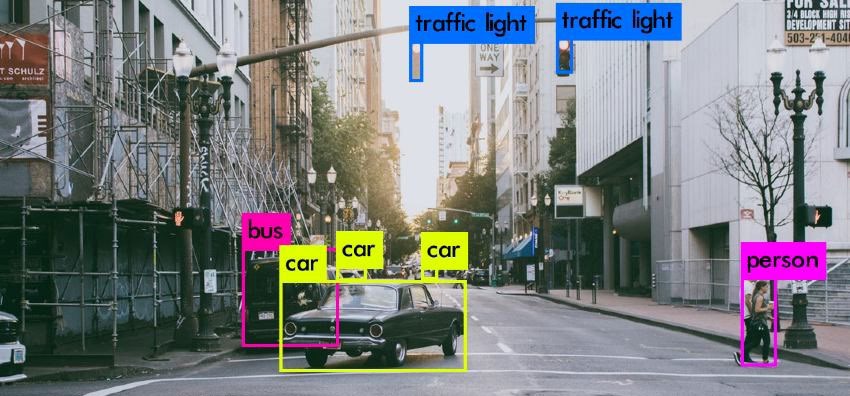
Just as oil refiners convert crude oil into gasoline and other refined products, data labeling startups such as Scale AI, Snorkel AI, and Weka play a critical role in refining data for AI model utilization. Positioned as the gatekeepers of mass AI adoption, these startups are set to attain significant value in the coming years, especially as enterprise SaaS companies are focused on integrating AI across their current and future product suits.
Scarcity of well-curated datasets has been a persistent challenge in AI and ML development, elongating development timelines and compromising accuracy. Without robust datasets, AI applications suffer from reduced functionality and heightened susceptibility to vulnerabilities. AI adoption is already picking up pace across the globe and data labeling startups are the gatekeepers as they curate the data for AI integration. 35% of global companies use AI, per Exploding Topics. 73% of US companies have already adopted AI in at least some areas of their business, according to the 2023 Emerging Technology Survey by PWC. Larger enterprise companies are 2x more likely to use AI than smaller businesses.
Data labeling startups play a pivotal role as facilitators of AI adoption. Despite the abundance of data in companies worldwide, it requires curation before being suitable for AI model training. The demand for AI data labeling is escalating with the surge in demand for application-specific AI models. Additionally, the increasing adoption of proprietary AI models, trained on internal databases, further drives the need for AI data labeling startups.
AI data management startups specialize in data labeling, a process of categorizing data using both software and a 'human-in-the-loop' approach. This transforms any database into one that is compatible with AI models. Training models on such curated databases can significantly enhance model outcomes. This enhancement extends beyond performance alone, impacting:
•Training process efficiency: Accelerating model training, enhancing performance, and reducing computational expenses, thereby boosting the productivity of ML teams.
•Model performance across all queries: Improving performance not only on common queries but also on the less frequent yet crucial queries, enhancing overall business impact.
•Model size optimization: Enhanced data quality leads to smaller, more portable models, reducing operational costs while maintaining performance levels.
Enterprise SaaS companies have been under pressure as reflected in the broader P/E trailing multiple of the sector in 1Q24 compressing 68% to 5.3x, and revenue growth hitting a low of 16%. Leveraging AI has the potential to bolster the financial performance of these firms, with enhancements in number of features including automation, predictive analytics, bolstered cybersecurity, scalability, and cost efficiencies. Gartner forecasts a robust 34% growth in AI adoption within SaaS, poised to reach an impressive $27 billion market size by 2025.
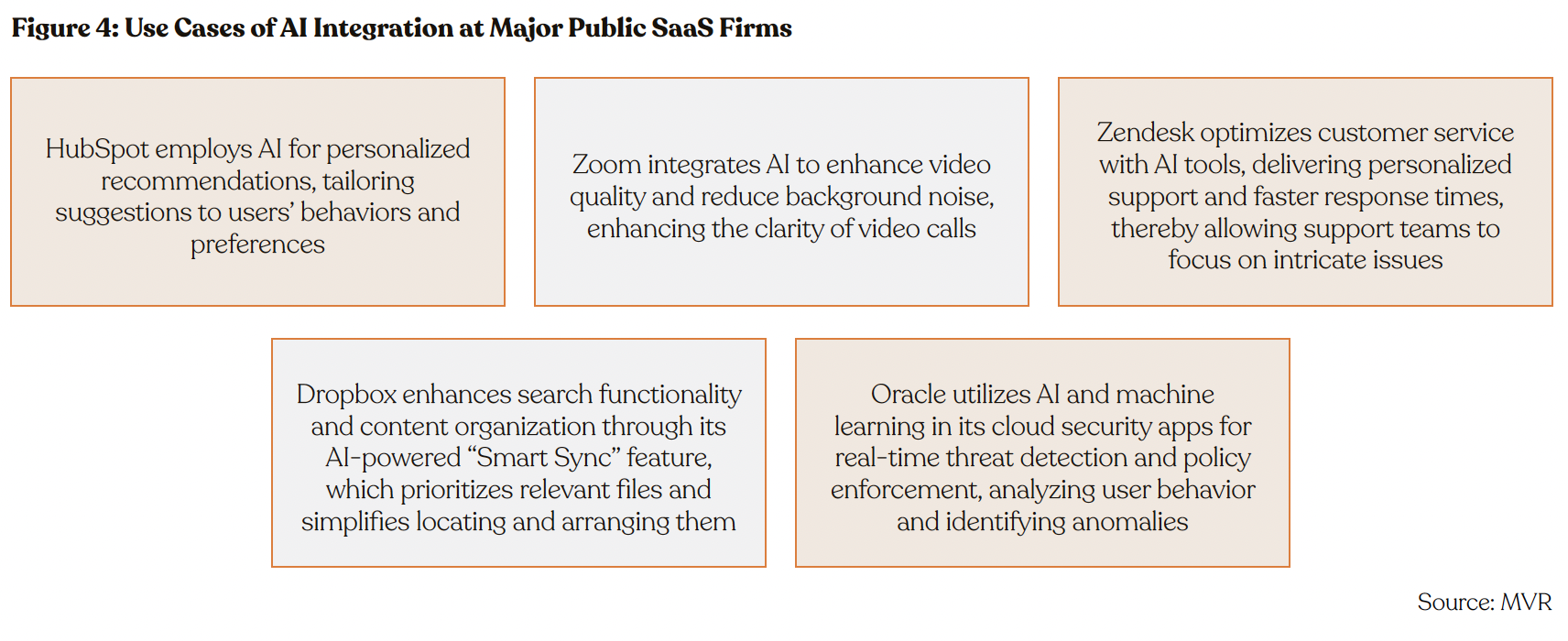
The global AI datamanagement market is projected to grow from $25.1 billion in 2023 to $70.2billion in 2028, at a CAGR of 22.8%, per MarketsandMarkets. AI data managementstartups such as Scale AI, Weka, Voxel51, Snorkel AI, and Superb AI, amongothers, are poised to become highly valuable companies in the future.
SnorkelAI, a California-based AI data management startup,has enabled companies like Chubb, BNY Mellon, and multiple government agenciesto expedite AI application development by 10x to 100x. A 467% valuation markupbetween series B and C rounds underscores investor confidence. Scale AI,a California-based data labeling startup, recently raised $1 billion at avaluation of $13.8 billion, an 89% markup from the previous round, underscoringinvestor’s confidence. Adoption by OpenAI, Meta, and Microsoft, among others cementscredibility. Weka, a California-based AI data management startup raiseda $100 million series E round at a valuation of $1.6 billion, up 113% from theprevious round. With revenue doubling annually and on track to triple in 2024,the startup has demonstrated a robust financial trajectory.

What’s a Rich Text element?
Heading 3
Heading 4
Heading 5
The rich text element allows you to create and format headings, paragraphs, blockquotes, images, and video all in one place instead of having to add and format them individually. Just double-click and easily create content.
Static and dynamic content editing
A rich text element can be used with static or dynamic content. For static content, just drop it into any page and begin editing. For dynamic content, add a rich text field to any collection and then connect a rich text element to that field in the settings panel. Voila!
How to customize formatting for each rich text
Headings, paragraphs, blockquotes, figures, images, and figure captions can all be styled after a class is added to the rich text element using the "When inside of" nested selector system.