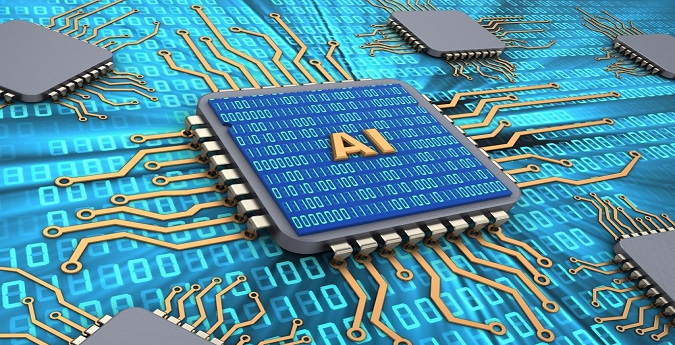
Venture Bytes #95: The Race for AI Hardware Supremacy Gets Intense

The Race for AI Hardware Supremacy Gets Intense
The rapid adoption of generative AI has intensified the AI arms race. While Microsoft and Google have been battling it out with their language models, a quieter yet equally significant rivalry is brewing on the hardware front between the big tech companies as well as some new innovative start-ups. With the global AI chip market size expected to reach $227 billion by 2032 from $16.9 billion in 2022, per Precedence Research, the AI hardware battle represents a critical step in the development of AI and its applications.
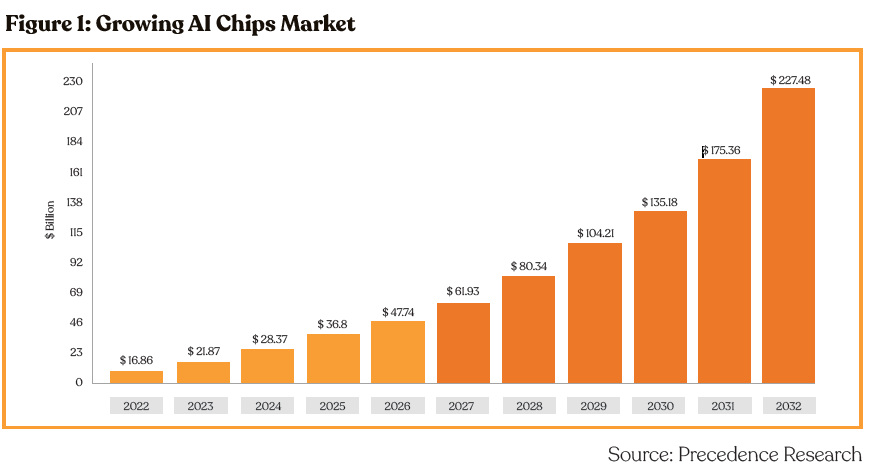
Growing GPU Demand Offers Opportunities
The explosive growth of generative AI has triggered a sharp increase in demand for graphics processing units (GPUs). According to prior projections by market intelligence provider Trendforce, the GPT model required approximately 20,000 GPUs to process training data in 2020. That estimate has now increased to over 30,000. Microsoft recently incorporated ChatGPT features into its Bing search engine. Should Google plan to integrate ChatGPT-like features into its search, it will require 512,820 A100 HGX servers with a total of 4,102,568 A100 GPUs, representing $100 billion in servers and networking capex, according to Semi Analysis. These numbers are indicative of the sheer scale of computational resources that are necessary to support the development and deployment of advanced AI models like GPT. Meta, another AI heavyweight, announced a shift from CPU-based to GPU-based models in its February 2023 earnings call, highlighting the increasing importance of GPUs in the AI ecosystem.
Gartner’s research suggests that the share of specialized chips such as GPUs used in data centers is expected to exceed 15% by 2026, up from less than 3% in 2020. Nvidia estimates that the need for processing power for most machine-learning or AI tasks has increased by 25 times every two years. With the growing dominance of generative AI, the demand for GPUs and specialized chips is expected to rise significantly, benefiting all participants in the value chain.
According to McKinsey, the demand for AI-related semiconductors is expected to grow at a rate of 18% over the next few years, five times higher than the rate projected for non-AI applications. By 2025, AI-related semiconductors could constitute around 20% of all demand, generating an estimated revenue of $67 billion. This rapid growth presents opportunities for semiconductor companies at data centers and the edge. If the growth rate remains consistent, semiconductor companies could capture up to 40-50% of the total value generated by the AI technology stack.
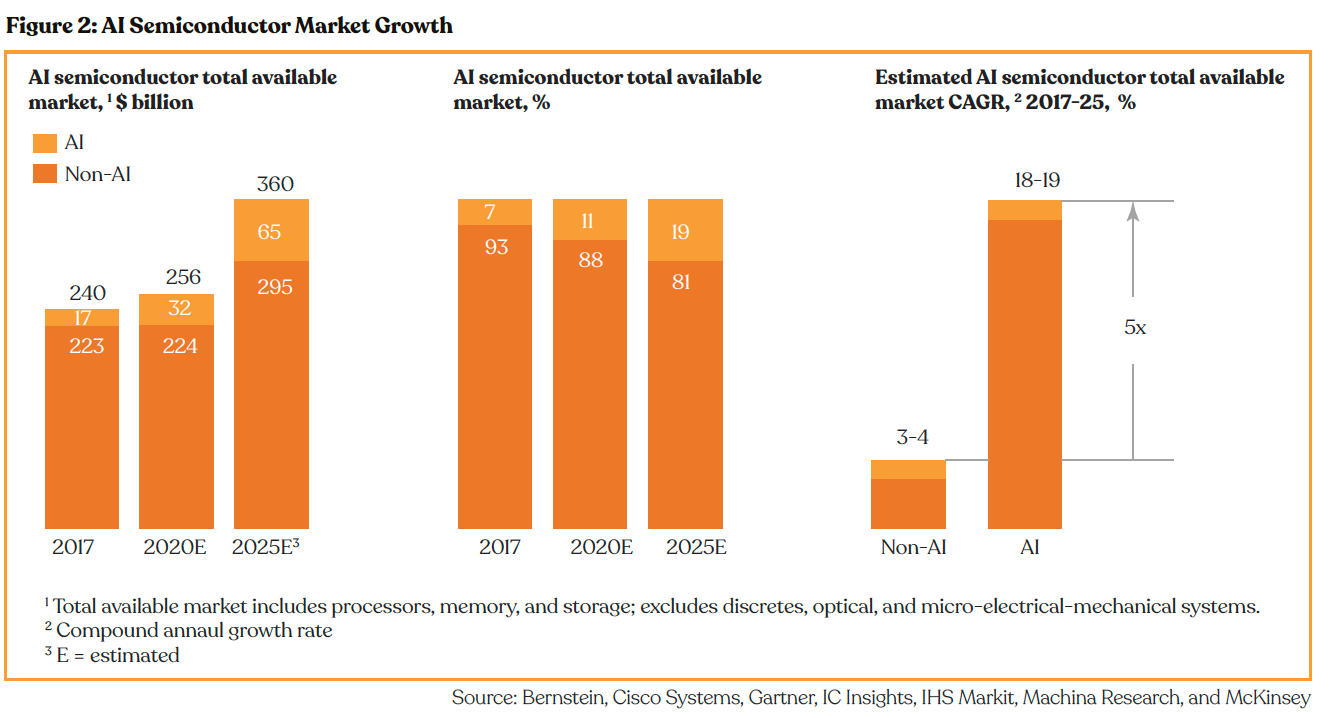
Big Tech Challenges NVIDIA’s Leadership
NVIDIA currently holds 90% of the market share in AI hardware, per New Street Research. At the recent NVIDIA GTC conference, CEO Jensen Huang reaffirmed the company’s commitment to large language models (LLMs) and their role in shaping the future of generative AI. To that end, Nvidia has introduced new cloud-based services for generative AI and supercomputing. These services will enable customers to purchase AI solutions directly from Nvidia, access pre-trained generative AI models, and harness the computational power of the company’s DGX AI supercomputers.
The world’s leading GPU maker is cashing in on the AI revolution but faces growing challenges from cloud hyperscalers who see chip projects as a critical part of their strategy to cut costs. Google, a leading player in the AI industry, has recently announced the launch of its latest AI supercomputer, which it claims outperforms comparable systems from NVIDIA in terms of both speed and energy efficiency. The new system, named TPU v4, is fueled by Google’s internally developed Tensor Processing Unit (TPU) - a specialized chip designed for AI applications that have been under development since 2016. TPU v4 delivers a performance boost of between 1.2x and 1.7x over NVIDIA’s A100 while consuming 1.3x to 1.9x less power, thus enhancing Google’s technological edge in the competitive AI semiconductor market
In response to the growing demand for AI-capable GPUs, Microsoft, which currently uses “tens of thousands” of Nvidia GPUs in its Azure platform, is reportedly joining forces with AMD to challenge Nvidia’s dominant position in the AI semiconductor space. This strategic move underscores Microsoft’s deepening involvement in the chip industry while posing a significant challenge to Nvidia’s near-monopoly in the market.
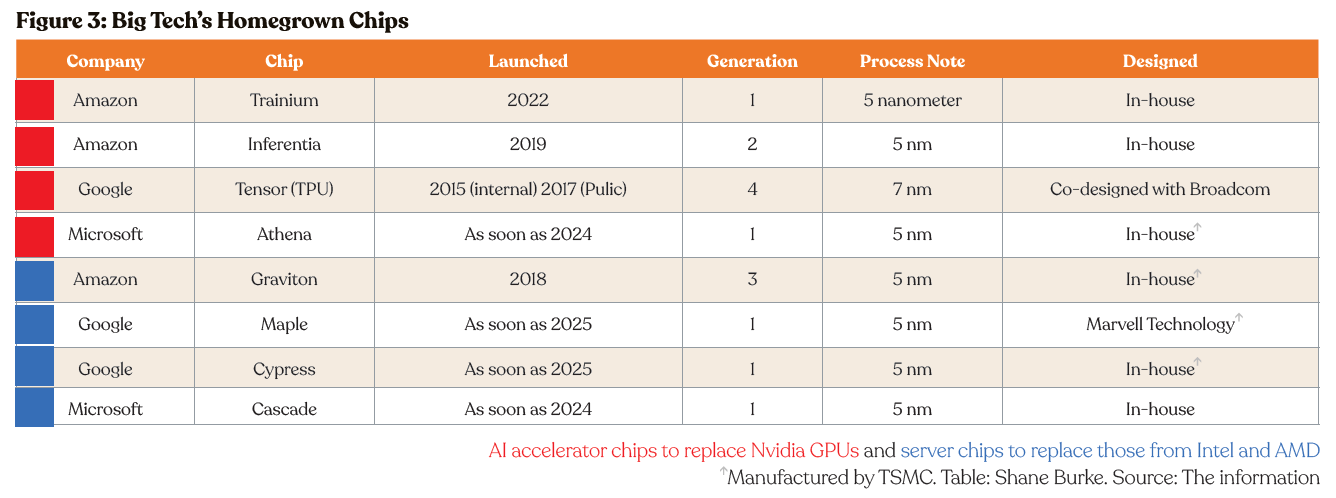
Tech behemoths are not only developing their own AI chips but are also forging partnerships with NVIDIA to enhance their AI capabilities. In November 2022, Microsoft unveiled a multiyear collaboration with NVIDIA to build a cloud AI supercomputer that enables enterprises to develop large language models and complex recommender systems. Meanwhile, Google and NVIDIA announced a joint venture in March 2023 to launch hardware instances that cater to AI-specific requirements. In addition, NVIDIA has partnered with AWS to build the world’s most scalable and on-demand AI infrastructure, tailored to train increasingly complex large language models and advance generative AI applications.
The competition between these big tech firms highlights the importance of hardware advancements in the rapidly evolving field of AI. As companies continue to push the boundaries of what’s possible with AI, it’s essential to have hardware that can keep up with the intense computational requirements of LLMs and other advanced AI applications.
Opportunities for VCs
As the market for AI hardware continues to grow, it’s likely that we’ll see new players entering the fray alongside established players like NVIDIA. This new wave of interest is expected to bring benefits to the participants across the supply chain for GPUs and AI chips. Accordingly, start-ups offering GPU cloud computing, AI accelerator chips, and other specialized hardware for AI are seeing an opportunity. New Jersey-based GPU-focused cloud compute provider CoreWeave, which recently landed a $221M Series B funding, provides access to 12+ SKUs of Nvidia GPUs in the cloud including H100s, A100s, A40s, and RTX A6000s, for use cases like AI and machine learning. The company, valued at $2 billion (pre-money) believes that the dominant cloud providers’ legacy cloud infrastructure is not suitable for generative AI. Similarly, Canada-based Arc Compute provides custom GPU cloud services and offers GPU chips from Nvidia and Intel Geospatial.
“There’s new openings for attack and opportunity for those players (VC-backed start-ups) because the types of chips that are going to most efficiently run these algorithms are different from a lot of what’s already out there,” - Brian Schechter, Partner, Primary Venture Partners
Founded in 2016, Cerebras Systems has been able to capitalize on the growing interest in generative AI. The company, which has raised $718M through six funding rounds, enables AI research at high speeds and scale. The company has partnered with AI pioneer Jasper AI as well as companies such as GalaxoSmithKline, TotalEnergies and AstraZeneca. GSK, for instance, uses its CS-2 system to train language models at a scale and size previously unattainable. California-based SiMa.ai, which raised a $30 million Series B-II round a year ago, is developing a system-on-chip platform for AI/ML applications to make ML deployment easy for clients.**
Venture Debt Gains in Prominence
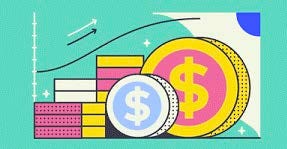
Venture debt has evolved over the past decade as a pragmatic funding option for startups. According to Pitchbook, the US venture debt deal value surpassed $34 billion in value across nearly 2,500 deals in 2022, representing an impressive 4.6x growth since 2012. Further analysis of Pitchbook data shows that venture debt has consistently accounted for around 10-20% of the total venture funding in the US in the last decade.

venture debt, with KKR expressing interest in underwriting loans to startups and Blackstone announcing $2 billion toward venture loans. These investments are part of a larger trend of private equity firms and other institutional investors moving into the venture lending market.
What’s Driving Venture Debt Growth?
Venture debt is increasingly becoming favored as an alternative investment option for startups to raise funds across all stages of growth in the current market conditions. Much like the wider venture capital (VC) market, venture debt has benefitted from affordable capital in the last few years. With overall VC funding slowing down and crossover investors pulling back, there has been a liquidity crunch in the market, resulting in a record-high ratio of capital demand-to-supply for late-stage companies in 1Q23. Pitchbook data suggests only 31 cents are available for every dollar demanded by late-stage VC-backed companies. As a result, US VC start-ups are exploring nondilutive options to extend their runway further, and venture debt has emerged as a favorable option for many companies. Despite a surge in interest rates, venture debt remains a practical option due to its flexible and non-dilutive nature. The high capital demand-to-supply ratio is also likely to lead to a more lender-friendly environment and a higher bar for debt issuance. That being the case, lenders are expected to prioritize metrics such as EBITDA and enterprise value to mitigate risk and address potential future losses.
Venture Debt in the Aftermath of SVB Collapse
The share of Silicon Valley Bank (SVB) in the total venture debt issuance of 2022 was $6.7 billion, or nearly 22% of the total issuance. Understandably, the collapse has left a void in the venture debt market, but this is an exciting opportunity for non-bank venture debt lenders, which generally charge a 3.25% prime interest rate and generally 6-8% interest. According to an industry analysis by venture lender Applied Real Intelligence, venture debt has consistently offered a 15-20% return over the last two decades. The Los Angeles-based lender also estimates the annual average losses for venture debt players well below 0.50%.
Given that many start-ups that raised their last equity rounds in 2021 must be in need of capital (assuming an average equity capital infusion lasts 18-24 months), venture debt could be a pragmatic option for start-ups looking to raise funds without a down round. Venture debt becomes an even more favorable option as the beaten-down valuations translate into a significant stake dilution.
“Over the past two decades, venture debt has consistently produced annual returns in the 20 percent vicinity with minimal losses, very low volatility of returns, and limited correlation to other assets. The current opportunity is the best we have ever witnessed given significant demand for capital and limited supply as VCs and banks scale back,” - Zack Ellison, Managing General Partner, Applied Real Intelligence
Despite a considerable amount of uncertainty surrounding venture debt in the aftermath of the SVB crisis, there exists a strong inclination among non-bank lenders to embrace venture debt financing, as evident from the foray of players such as AllianceBernstein and Centerbridge, among others, into this fast-emerging asset class. Further, with strong underlying demand for venture debt and higher participation from varied capital providers, we expect the venture debt market to gain further prominence despite a slow start to 2023.**
What’s a Rich Text element?
Heading 3
Heading 4
Heading 5
The rich text element allows you to create and format headings, paragraphs, blockquotes, images, and video all in one place instead of having to add and format them individually. Just double-click and easily create content.
Static and dynamic content editing
A rich text element can be used with static or dynamic content. For static content, just drop it into any page and begin editing. For dynamic content, add a rich text field to any collection and then connect a rich text element to that field in the settings panel. Voila!
How to customize formatting for each rich text
Headings, paragraphs, blockquotes, figures, images, and figure captions can all be styled after a class is added to the rich text element using the "When inside of" nested selector system.